Money is pouring into AI businesses that promise cool innovations – but basic research needs steady public funding to solve the societal challenges that AI poses, argues University of Bergen researcher
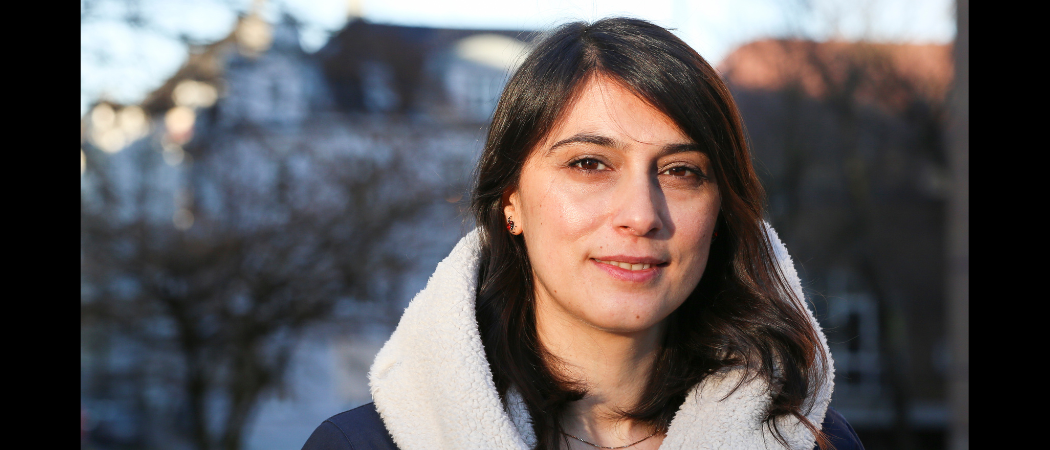
Marija Slavkovik, professor and head of the Department of Information Science and Media Studies at the University of Bergen, Norway.
In today’s global gold rush to develop artificial intelligence, a lot of the funding has been going to business innovation rather than early-stage research. That’s a mistake: AI business cannot exist without AI research. And unless funders keep the research going strong, the future of AI could prove a lot less interesting than expected.
To see what I mean, you only have to look back at 2023, the year of generative AI. Public attention was riveted on ChatGPT and Large Language Models in general. These systems built on years of publicly funded computing and AI research; and most were brought into the marketplace in 2023 with the help of large tech companies in the US. In Europe, policy makers got rattled, pledging huge sums to catch up in Germany, UK, France, Spain, Norway and the EU. But much of this money has been earmarked for innovation, not research. That’s because policy makers keep confusing AI research with AI business. So here’s an explanation of the difference.
Research v business
AI research is the pursuit of knowledge about computation to accomplish tasks that (the way a human does them) require intelligence to be accomplished. The “artificial” in AI is like the first word in “artificial sweetener”: you taste sweetness, but the substance that causes it is not sugar; you can’t make caramel out of it. In AI we are not creating intelligence with computation; we are creating tools that relieve the need to use intelligence. Just as there are many substances that can be used as artificial sweeteners, so there are many ways to accomplish an intelligence-demanding task. Some sweeteners are poisonous; and some AI might also end up being harmful for humanity. In research we are allowed to ask if we are currently investigating the right kind of AI.
But what is AI business? In the midst of trends, hype and branding, there is at present no common understanding of what AI business is actually about. The start of this latest wave in computing technology can be traced quite a way back, to 1997 when IBM’s Deep Blue defeated world chess champion Garry Kasparov. Then in 2011 IBM’s Watson defeated human champions in Jeopardy. Then came computers defeating humans in Go, poker, Space Invaders, StarCraft 2 and other games. Were these marvelous breakthroughs? No. They were privately funded advances supported by digitalisation (more data) and the commodification of computational processing power. The same dynamic was behind spam filters, text auto correction, map journey plans and the mid-2000s Google launch of its automated translation service.
And what is an AI business model? Is an AI application a product that the companies who develop it make money from selling? Not directly. Google chooses to give away its language translation service for free. Instead, Google’s main revenue stream is advertising: it uses AI to attract attention that can be sold. Likewise, IBM makes money only indirectly from its AI developments; instead, it sells software, infrastructure and consulting. OpenAI, the developer of ChatGPT, has not quite monetised its most famous product – but of course, it’s thinking about that.
So if AI isn’t actually generating cash for Big Tech, what will happen when their developers hit a big research obstacle? They will abandon it. We know because it already happened in what is sometimes called the first and second “AI winter” – periods when private funding and industry growth slowed. But while the private money dried up, the public funding continued. The AI we see today is a result of that sustained research.
The big research questions
Sustained research is what we need if AI is to advance further. Consider the example of language technologies. Large Language Models (LLM), the Oz behind the ChatGPT wizardry, works kind of great in English because the Internet works kind of great in English. ChatGPT was trained on texts from the Internet; and over half the resources on the Internet are in English. Spanish, by contrast, has a tenth of the English Internet footprint. Is there a strong correlation between the language of the text and what the text is about? Of course there is; if there weren’t, we could have used automated translation and an LLM trained on English data to answer Spanish questions. So it follows that to have a great Spanish ChatGPT you need an LLM trained on Spanish data.
But how can that work, if you start training LLMs in more and more languages? Building ChatGPT the way OpenAI did it is expensive, financially and ecologically. Can everyone afford the cost of preserving their cultural heritage via LLMs? Surely there’s a better, cheaper, greener way to build language-specific AI. But that is a question for fundamental research, not product development. And so far no one knows how to answer it. However, if you did solve it, you would get a much better business than OpenAI currently has.
There are many other examples in which Big Tech uses AI, hits a knowledge limit and makes shortcuts that have a range of unpredictable social impacts. Visual spatial mapping of robotic cleaners does not know how to exclude private information from its data. Data for AI is only useful if cleaned by people. Automated content moderation is not sensitive to context. We do not know how to get computer programs to preserve basic human rights, when they move far faster than a human can monitor them for violating such rights. And we do not know how to innovate our legal systems to handle the unclear jurisdictions that data and data processing impose.
These kinds of problems need deep research to solve. That requires sustained, ample public funding for support. Don’t misunderstand me: it is good that AI-based businesses get the private funding they need to develop products society needs. But to get AI business in the future we need to spend public funding on AI research in the present.
Marija Slavkovik is a professor and head of the Department of Information Science and Media Studies at the University of Bergen, Norway.