Spotting artificial intelligence-generated fake articles that threaten to undermine the scientific process is a joint responsibility. All researchers can play a part by making time to read research intelligently - as if reading a great novel
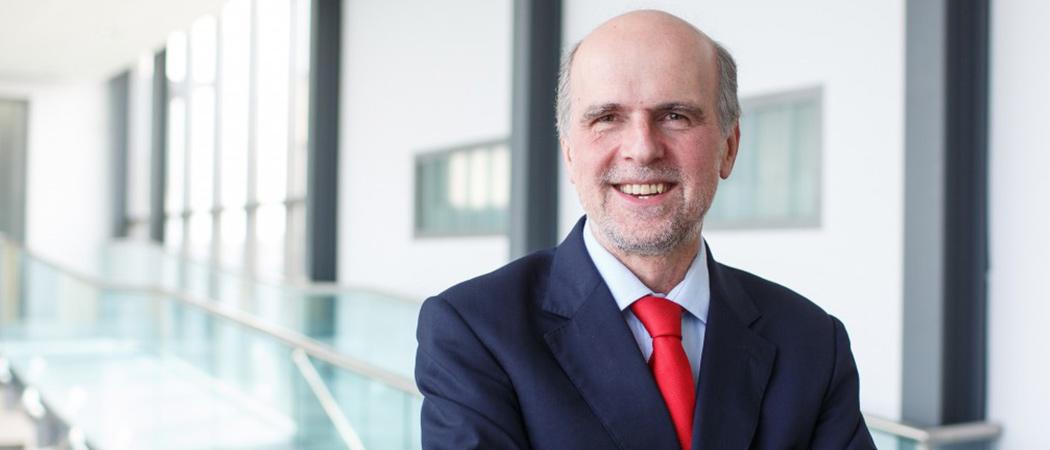
Prof Phillip De Wilde by University of Kent
Artificial intelligence is making our lives easier, and for unscrupulous scientists, it could make work a lot easier too, by generating research papers.
Science|Business recently reported that US lawmakers are increasingly worried by fake journal papers. Publishers are worried, too. So am I. Am I competing for grants with academics whose curriculum vitae are padded with fake papers, or who are rising up the citation indices because they are getting cited by fake papers?
It’s common knowledge that AI systems can now generate fake essays on any topic. These so-called transformers are part of the toolbox of natural language processing, that also provides help with translating documents, summarising text, and answering questions about it. And that’s useful.
Other AI software such as DALL-E can generate images. Do you want a picture of astronauts playing golf on Mars? AI can generate one for you.
All these AI systems are trained on lots of data. The data are transformed, and noise is added. That noise is essential if the system is to deal with data it hasn’t been trained on. When the system generates a new piece of text or a new image, it will use its data, but in a somewhat noisy way.
These systems are dubbed stochastic parrots, in other words, they stitch together and parrot back language based on what they've seen before. The actual systems are very sophisticated, and the data are often scrubbed or rebalanced before being used. In short, AI systems generating text or images are amazing pieces of software.
So, for example, a fake paper referred to in the Science|Business article starts as follows: “It has been observed that there is a plentiful emanation of neutrons from uranium (U) under the activity of slow neutrons. It is important to determine whether and how much the quantity of neutrons discharged surpasses the number retained.”
We have all read authentic, peer reviewed papers written in the same style. But those who know some physics will notice that the words ‘emanation’ and ‘discharged’ are used in an unusual way here.
Also, the convention is to name the specific isotope of uranium, and not just give the chemical symbol U.
AI generated text versus great works of literature
The ability of natural language processes to read and parse legitimate papers means that on first glance fake papers often sound plausible. Delve deeper and it is likely the logical deductions in a paper are either poor, or are very familiar. The veneer of coherence comes from the words and sentences, not from the logical structure. That is no surprise, given the core of current AI is stochastic and so rather than based on mathematical logic, is random.
Compare this to a great work of literature. In the first sentence of ‘The Brothers Karamazov’ the author Fyodor Dostoyevsky sets the scene for the entire plot of his deftly-crafted, 840 page book:
“Alexey Fyodorovitch Karamazov was the third son of Fyodor Pavlovitch Karamazov, a land owner well known in our district in his own day, and still remembered among us owing to his gloomy and tragic death, which happened thirteen years ago, and which I shall describe in its proper place.”
The opening sentence presages the whole structure of this immense novel. The sentences that follow all play a role in the logical unfolding of the story. A stochastic parrot can readily generate a novel in the same style, but the story will feel random. The narrative will not draw you in, as you would expect from a great novel, or indeed, a great research paper.
Although generated through natural language processing of the scientific literature, there may be no obvious, in-your-face plagiarism in a fake paper. On the other hand, anything new that is discussed is likely to feel slightly random. If there are figures, they may look familiar as well.
I’m sure, you like me, have read many papers, and even grant applications, that had the same feel. Surely, they weren’t all fake?
Picture the scenario of a researcher who is under pressure to produce a paper. The funding on the grant is about to run out. The experiments to date have all been negative. Desperately scanning through many papers our scientist finds related research with encouraging data. That provides the basis to put a more positive spin on the discouraging results. There may be little logical detail, but overall, the paper is planted in familiar scientific territory.
The head of the lab, who is writing the next grant application, spends 10 minutes looking at the paper, finds it is plausible, adds his or her name, and authorises the paper for submission. The reviewer for a conference spends another 10 minutes on the paper and accepts it.
This may seem an extreme example in some respects, but I think it will resonate for many. It is in essence human stochastic parroting, in which there is no overt plagiarism, but under pressure things find their way into a paper that are close in content to what others have written.
It also begs an important question: is a paper or grant application that sounds familiar to the reviewer more or less likely to be approved than a completely original masterwork?
Rooting out fake papers is important and all researchers can play a part in this by making time to read research intelligently - as if we are reading a great novel.
Don’t just scan the material for what is familiar but follow the story from beginning to end. Let’s read, write, and review less, but better. Whether it’s generated by machines or humans, this is the only way to avoid not just what is fake, but also what is simply flawed.
Phillipe De Wilde is professor of Artificial Intelligence in the Division of Natural Sciences, University of Kent, Canterbury, UK.