EU-funded ATTRACT consortium explores how to enrich MRI scans with mixed reality headsets and other high-tech wizardry.
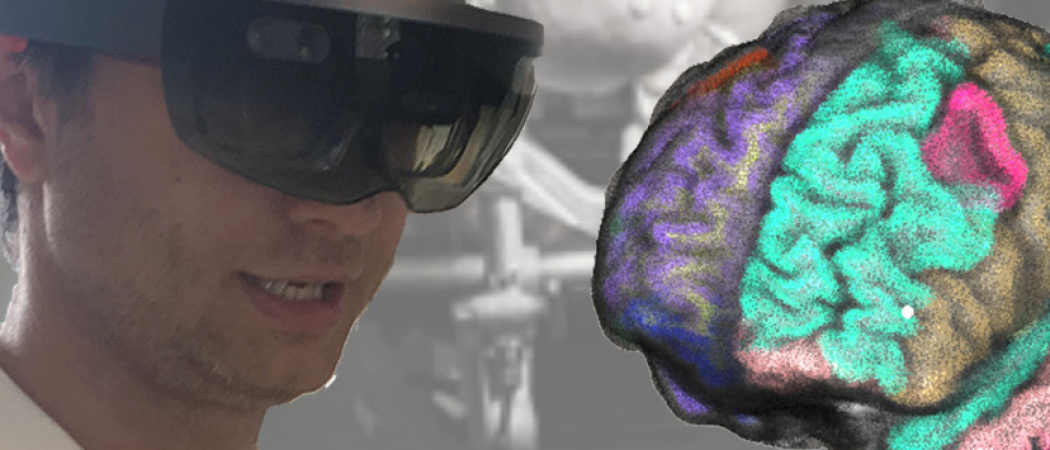
Copyright: MRBrainS
The complexity of the brain makes operating on this organ one of the most challenging tasks in medicine. But a group of Italian researchers is trying to make intricate neurosurgery easier with holographic brain-mapping software, which highlights and labels crucial areas and blood vessels right before the surgeon’s eyes.
Their project, called MRbrainS, feeds brain activity data from functional magnetic resonance imaging (fMRI) into dedicated software for brain-mapping (called a neuronavigator), then integrates that into a mixed reality headset, which overlays 3D digital images on to the wearer’s view of the real world. These images can be linked to objects in the real world and remain anchored to them as the wearer looks around.
MRBrainS is one of eight research projects focused on magnetic resonance imaging (MRI) with support from ATTRACT, a €20 million consortium funded by the EU and led by CERN that has awarded €100,000 each to 170 technology projects. First developed in the 1970s, MRI is a medical imaging technology that uses magnetic fields and radio waves to create detailed images of internal organs, tissues and bones.
Today’s neuronavigators display 2D images on screens, forcing the surgeon to mentally link what’s on the display with the patient lying on the operating table. That’s difficult and “slows down the whole procedure,” says principal investigator Antonio Ferretti. But using a mixed reality headset to tie 3D information directly to what surgeons see in front of them means they can rely on hand-eye coordination, “which is easier if your hands are in front of you, in the same direction you are looking,” explains Ferretti.
Another problem MRbrainS aims to solve is that today’s neuronavigators don’t incorporate fMRI data. Unlike regular MRI or computed topography (CT), fMRI shows activity in different parts of the patient’s brain, something that previously could only be determined during invasive surgery with targeted electrical pulses, called direct cortical stimulation. The different steps necessary to push fMRI data to a neuronavigator currently require various different software packages, most of which are designed for researchers, not surgeons. Turning the neuronavigator’s output into a mixed reality image is yet another challenge.
For now, MRbrainS uses the Microsoft HoloLens mixed-reality headset. But Ferreti said a longer-term goal could be to design a new headset that integrates the feed from a surgical microscope with all the other data sources in a single device. “This is why we hope that in future development we could involve larger companies,” says Ferretti, an assistant professor of physics at Annunzio University’s Institute for Advanced Biomedical Technologies (ITAB), which runs MRbrainS in partnership with the University of Pavia startup SerVE.
Operating inside the womb with mixed reality
Similarly, the ATTRACT-funded MIFI project is developing a mixed reality system that integrates MRI, ultrasound, and endoscopic video for surgery on unborn children. In-utero surgery is especially difficult, because doctors “need to operate on a patient inside another patient,” notes Mario Ceresa, MIFI’s principal investigator. That patient is very small and delicate, and depends on an amniotic sac that can quickly collapse, so “the interventions are very difficult, because there is a lot of time pressure,” adds Ceresa, a postdoctoral researcher at Pompeu Fabra University (UPF) in Barcelona. Another challenge is that since the operation is keyhole surgery, the surgeon has only a very narrow field of view inside the womb through an endoscope, a tiny camera on the end of a long, thin fibre optic cable.
MIFI aims to improve the surgeon’s field of view by displaying a virtual 3D image of the mother’s womb in mixed reality, on top of what the doctor sees in front of them. The project applies machine learning to pre-operative ultrasound and MRI scans to identify relevant blood vessels—some of which are extremely small—and to help the surgeon find them in the womb, even if the baby has moved in the meantime.
Though the system isn’t meant to be specific to one condition, for development purposes MIFI is focusing on surgery to correct a condition called Twin-to-Twin Transfusion Syndrome (TTTS). This is where twins that share a placenta — monochorionic twins — are threatened by a blood flow imbalance; the condition occurs in one third of monochorionic twins, and kills both in more than 90 per cent of cases. TTTS can be treated by separating the twins’ blood circulation, using a laser to coagulate the tiny blood vessels. MIFI is a partnership between UPF, research firm Vicomtech, and Sant Joan de Déu Hospital in Barcelona.
Finding scars fast
MERIT-VA is another ATTRACT project trying to improve the way major surgery is carried out. The researchers, based at the Teknon Medical Centre in Barcelona, UPF, and software firm Galgo Medical, are using machine learning to analyse data from MRI scans and electrocardiograms (ECGs) to improve planning of a particular type heart surgery.
Scar tissue formed after a heart attack can disrupt the heart’s natural electrical pulses by directing the current where it shouldn’t go, causing an irregular heartbeat (arrythmia). The condition is treated by inserting tiny catheters into the heart that destroy the problem tissues with radio waves. These catheters contain sensors that provide their position in 3D and detect electrical signals to identify the tissues that need removing. This information can then be displayed on an electro-anatomical map (EAM) to guide the surgeon.
But building this map using the catheters can take hours, increasing the risk that something will go wrong during surgery. The condition also frequently recurs after treatment. The more the surgeon knows about which scars to target and where to find them, the quicker the procedure and the greater the chance of curing the condition without destroying excess tissue unnecessarily.
Doctors can predict where in the heart the problem is likely to be found by looking at ECG charts, and MRI has been shown to improve this pre-op planning. MERIT-VA, therefore, aims to improve such predictions further by using machine learning to analyse and integrate ECG and MRI information. The goal is to make the surgery quicker, less risky, and more successful.
In another project, called QP-MRI, researchers at the University of Turin and the University of Aberdeen are using a variable-field strength MRI scanner to monitor the structural integrity of a new type of medical implant. The implants, used to repair bodily tissues, such as bone, cartilage or corneas, are made from a biodegradable polymer lattice, bonded to an amino acid called polyhistidine, which shows up brightly in MRI scans. When the lattice begins to break down, the MRI signature of the polyhistidine fades.
The lattices are supposed to break down once their job is done, but the point is to ensure they don’t deteriorate too early. Such polymer lattices are already in medical use; QP-MRI’s novelty is the use of polyhistidine as a contrast agent, along with an MRI scanner capable of operating at variable magnetic field strengths, designed by the team at Aberdeen.
“Our system uses a completely new mechanism in order to produce contrast in an MRI machine,” says principal investigator Simonetta Geninatti Crich, a professor of molecular biology at Turin. Geninatti explains the existing MRI contrast agents carry health risks, which is why polyhistidine is a desirable alternative. But in order for it to work, new, lower-field strength scanners are needed. “You can detect this signal only if you are able to work at a low magnetic field strengths of about 30 milliteslas, more or less,” whereas conventional MRI scanners work at around 1 tesla, she adds.
Machine learning dissects the detail
The MAGRes project aims to make MRI more effective at monitoring glioblastoma—an extremely aggressive form of brain cancer—by identifying subtle variations in MRI scans. The MAGRes researchers use magnetic resonance spectroscopy imaging (MRSI) to identify metabolic changes in the tumour. They then link these results to barely-perceptible changes in ordinary MRI scans, in order to develop new machine learning models for analysing MRI. The idea is not for glioblastoma patients to undergo MRSI—which takes much longer than MRI—but for MRSI research to make MRI analysis more effective.
“This metabolic information can appear before anatomical information seen by MRI,” explains Ana Paula Candiota, MAGRes principal investigator and postdoctoral researcher at the Network Centre for Biomedical Research and the Autonomous University of Catalonia. The hypothesis is that “we can use the metabolic information to try to guide ourselves to find things on the [MRI] image that maybe we did not know,” she adds.
The purpose of MAGRes is to detect as early as possible whether a patient’s treatment is having any effect or whether it needs to be changed, since glioblastoma patients are tragically short on time. Average life expectancy with treatment is a little over one year, and only a small percentage of patients survive five years.
Candiota says she also took part in experiments that eliminated glioblastoma in half of affected mice, without recurrence, by reducing the frequency of chemotherapy treatment to give the immune system more time to attack the tumour. But trying this method in humans is difficult because doctors and patients are suspicious of the hope of getting better results from less chemotherapy, she warns. The only human trials so far involved patients who “were in the last days” and had failed to respond to any treatment, so in all likelihood, nothing could be done for them. “That’s not fair,” notes Candiota.
In a similar vein to MAGRes, the IMAGO project aims to develop new models of MRI analysis using a technique called single particle tracking (SPT) to monitor the behaviour of light in sample tissues. Unlike MRI, SPT can identify tiny, sub-microscopic features, but MRI can “see” inside the body whereas SPT can’t. The IMAGO experiments aim to link the characteristics of different samples to subtle variations in MRI data, so that more information can be gleaned from MRI scans. The project is a partnership between Italy’s National Research Council and the Sapienza University of Rome.
Meanwhile, the DentMRI project is using low-strength MRI scanning to improve dental care, by providing the first ever images of teeth and gums together that are good enough for medical diagnosis. The researchers, based at the Polytechnic University of Valencia and MRI equipment manufacturer Tesoro Imaging, have developed a prototype scanner that can accommodate objects of up to a cubic centimetre, and the goal is to build one large enough for a person to put their head inside for a dental scan.
Enabling electronics at extreme temperatures
The Low Temperature Communication Link (LTCL) project could help to make MRI equipment more efficient by redesigning the way the powerful magnets inside an MRI scanner are connected to the rest of the system.
MRI magnets are kept cool with liquid helium, which has a boiling point of -269° Celsius, or about four Kelvins. Normal electronics can’t function at such low temperatures, so they are built outside the cryogenic vessel that contains the magnets and connected with wires. But LTCL aims to develop electronics that could work inside the cryogenic container, with a wireless communications link and wireless power supply to the normal temperature environment outside.
The LTCL researchers at CERN, startup Oxford Instruments, and the French Alternative Energies and Atomic Energy Commission (CEA) say this would bring a number of advantages—not just for MRI, but for any technology that relies on cryogenic equipment. For example, engineers would have more freedom in how they design the cryogenic containers because there would be no need for physical connections to the outside. Furthermore, bringing the electronics closer to the data source—the magnet—would cut interference and give more accurate readings.
Discover more ATTRACT projects developing MRI technologies and innovative solutions for society here. Also, save the date for the ATTRACT online conference - Igniting the Deep Tech Revolution.