Some experts are sceptical about the application of AI to employment practices
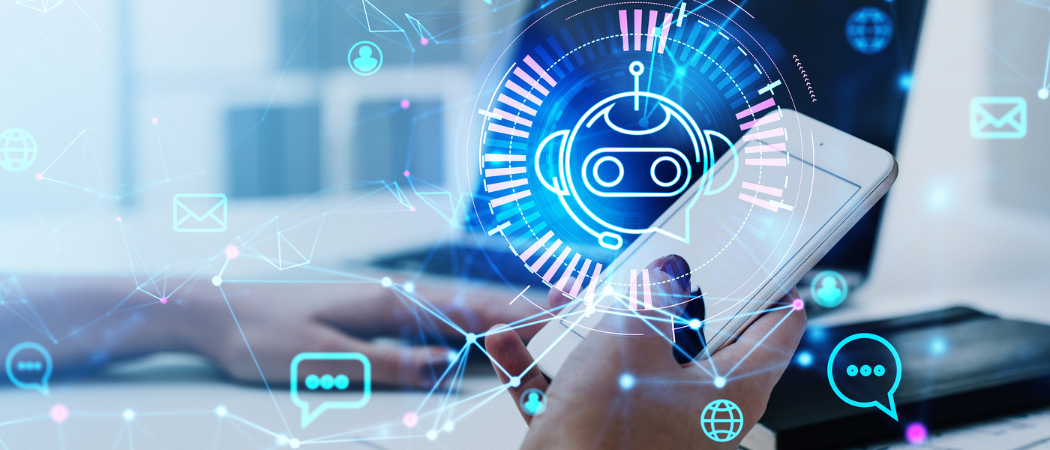
The digitisation of workplaces is generating vast amounts of data that could, in theory at least, be used to better manage employees. That data can be used to train algorithms to perform a number of management-related tasks, such as assessing candidates’ and employees’ talents, capabilities and performance, matching workers to tasks and/or clients, and even gauging whether an employee is close to burn out.
However, experts believe employers should proceed with caution, noting that there is scant evidence that automation is improving employment practices. As AI systems learn from historic data, there is a risk that they simply automate past mistakes, such as a bias towards male recruits, and end up replicating them on a larger scale. “It won't suggest improvements to reflect a changing context, such as a pandemic,” warns Matissa Hollister, a professor of organisational behaviour at McGill University in Montreal. “It's a cutting edge technology that encodes the status quo. It will pursue a pre-existing outcome, which it will seek to maximise, come hell or high water.”
A report published in May 2022 by the European Parliamentary Research Service Scientific Foresight Unit was even more blunt. “One of the biggest problems is that, in reality, most of these tools do not work,” it says, arguing that AI isn’t yet sophisticated enough to deal with all the nuances involved in managing people. “Some tools might show too many false positives or negatives,” the report notes. “For example, it may falsely identify someone who is at risk of burnout while, at the same time, neglecting a person who truly needs help but does not receive it since the AI is not picking up on the right signals.”
Indeed, the draft EU AI Act classifies employment as a high-risk application of artificial intelligence and proposes various safeguards, such as “conformity assessments” and mandatory disclosures. For example, an employer would need to inform an employee that they are being analysed by an emotion recognition system.
What are we trying to optimise?
Today AI systems are widely used in the so-called gig economy to allocate specific jobs, such as a food delivery, to the nearest available contractor. Such systems could also be used in-house by large employers, but there is a risk that they will only look to optimise short-term productivity, rather than the long-term health of the organisation and its employees.
Applying just-in-time scheduling and assessment software to manage employees’ time is fraught with pitfalls, notes Hollister, because the AI system can’t possibly know what is happening in an individual’s life and whether they are actually the right person to perform a specific task at a particular time. A good human manager, by contrast, will be sensitive to employees’ personal circumstances and can allocate and assess tasks accordingly. “In the long term, there are major headaches in terms of employee relations, of reputation, and productivity, and burnout,” warns Hollister. At the same time, some employees may try and “game” scheduling and assessment algorithms by focusing solely on the criteria they use, and neglecting other potentially important aspects of their roles.
Indeed, one of the fundamental challenges with applying AI to employment practices is the sheer number of variables involved. “Optimisation is a process based on a few variables from a set of many variables, so the ones who choose the variables, bias the process,” notes Ulises Cortés, a professor of AI at the Universitat Politècnica de Catalunya. He points out that even if an AI system manages to balance the interests of the business plan and the employees, it will likely fail to take into account other important considerations, such as environmental impact. “Optimisation can be perfect, but only from one point of view,” he warns.
Moving beyond big data
One way to mitigate this challenge is to build more complex AI systems that take many more variables into account. Yet collecting as much data as possible from as many sources as possible may be a waste of time, money and energy. Worse still, collecting lots of data about your employees could damage your reputation.
Cortés says that some companies, such as some of the major U.S. tech players, are no longer trying to collect as much data as possible. They are now more selective. “If you are 30 minutes in the loo, but you are performing well, who cares?” he asks. “Until the context changes, you don't need to retrain and then collect and curate the data. There's no magic in just collecting data,” adds Cortés. “If you are seen as the one that is collecting all the data from everyone, you are big brother. You are not a good guy.”
Some experts have questioned whether it is really necessary to implement AI at all in an employment context. “Will the introduction of AI into various institutions and workplaces across society really lead to prosperous, thriving societies as is being touted?” asked Phoebe Moore, now a professor of management at the University of Essex Business School, in a paper she wrote for OpenMind BBVA in February 2020. “Or will it deplete material conditions for workers and promote a kind of intelligence that is not oriented toward, for example, a thriving welfare state, good working conditions, or qualitative experiences of work and life?”
Yet many HR departments, particularly those that are inundated with job applications, continue to experiment with AI systems. Given the risks involved, Cortés of the Universitat Politècnica de Catalunya argues that these systems should be audited by third parties that can make binding decisions to correct errors or flaws. However, he says the auditors should have to sign non-disclosure agreements as employers’ AI systems could be based on potentially valuable intellectual property.
Aware of the limitations of AI, some companies have gone back to prioritising the recruitment and retention of qualified workers over automation, according to Cortés. In some cases, there is a growing recognition that you need people that your customers can relate to. “Some of these companies are looking is to hire more local people in their vicinities because that gives more credibility to the kind of products they are selling,” he adds.
Will AI earn our trust?
Of course, well-designed AI systems could help employers recruit and retain staff that are aligned with their values and those of their customers. For Shuai Yuan, a researcher in leadership and management at the University of Amsterdam, the extent to which AI systems are used for recruitment will depend on whether we begin to trust AI in other contexts, such as diagnosing illness or landing airplanes. “A lot of things in life have already been replaced or facilitated by AI,” he notes. “If we build up a certain trust with AI, we won't see that there is a huge problem with AI making these important decisions.”
Noting that AI systems are becoming more and more capable, Yuan contends that it should eventually be feasible to automate the entire recruitment process. “I really think everything can be done with AI as long as you give a very clear instruction as to what should be measured and what are the weights of the different measurements,” he says. “You don't need the human beings to really sit in this process. It’s not there yet, but I see that it is happening very fast.”
At the same time, Yuan believes AI systems used for recruitment or performance assessment will need to be audited by third parties for bias. As they film job interviews, employers could, for example, use emotion recognition software to assess a candidate’s personality. But this software needs to take into account that “people of different ethnicity or different races use different muscles and have different facial expressions,” Yuan cautions. “I'm from an East Asian culture, so we don’t smile that widely, which could be used to see whether you are confident person or not. There's a lot of this kind of potential discrimination involved in this process.”
Who checks the AI is doing its job?
One of the challenges will be finding auditors with the necessary expertise to understand how AI systems work and the significance of that in an employment context. “Who checks is a very important question because usually the algorithms are provided by the company that sells them, but then you have all this sensitive company-level data that goes into those algorithms, so they are proprietary as well,” notes Almasa Sarabi, an
assistant professor in leadership and management at the University of Amsterdam. “HR, for example, in most cases, does not really have the education or the knowledge to look at the algorithm and decide whether it is fit for purpose.”
Of course, human beings are also susceptible to discrimination and bias. There is a risk that an interviewer will be swayed by personal chemistry (or lack of it) with an interviewee. Sarabi believes this is an area where technology can make a difference. “We know from research that formalisation or standardisation of personnel practises really helps with generating more equitable outcomes, especially in interview situations where subjectivity can really creep in and you never really know what questions have been asked to whom,” she says.
Sarabi suggests that technology could be used to make the recruitment process more transparent and accountable by tracking which questions have been asked in an interview and logging the answers. However, she cautions against giving an AI system too big a role. “I don't think that AI actually needs to sit in and be part of the interview process as such and come up with its own questions,” she says.
Will there be rigid rules?
Once the EU AI Act is finalised, employers in Europe should have greater clarity over the extent to which they can harness AI. But it is not clear whether other jurisdictions will follow suit. To date, the US has taken a more laissez faire approach to AI than the EU. Although the White House has recently published an AI Bill of Rights, Hollister at McGill University, likens this document to a corporate mission statement, rather than a concrete set of rules that will actually change behaviour.
In the U.S., there are calls for regulators to take a step back because they lack the requisite knowledge to effectively regulate AI. A recent paper published by the University of Miami Law Review, co-authored by Keith Sonderling, a Commissioner on the U.S. Equal Employment Opportunity Commission, argues that “the most effective solution is a deregulatory approach that properly utilises the existing employment discrimination framework and the resources already available to agencies. Existing legal mechanisms that can help reduce the risks associated with AI should be prioritised without stifling innovation, even in the face of AI’s distinct challenges. To this end, self-regulation and self-audits should be encouraged and incentivised.”
Even so, some US states are introducing local regulations. And there is an argument that regulators need to try and get ahead of this challenge before AI systems become deeply embedded in employment practices and potentially encode serious flaws. For Hollister, AI systems should be employed as a scalpel rather than a sledgehammer – they should be used to address very specific failings rather than for the wholesale automation of recruitment and assessment. “There's this illusion that AI systems are objective and they absolutely aren't, because they're learning from patterns in past historical data and are capturing things that are problematic,” she stresses.