In medical image analysis, AI can surpass human experts; in diabetes it can raise an alarm in time to head off a glycaemic event. Hints of its power, but concerns about privacy are slowing deployment of AI tools
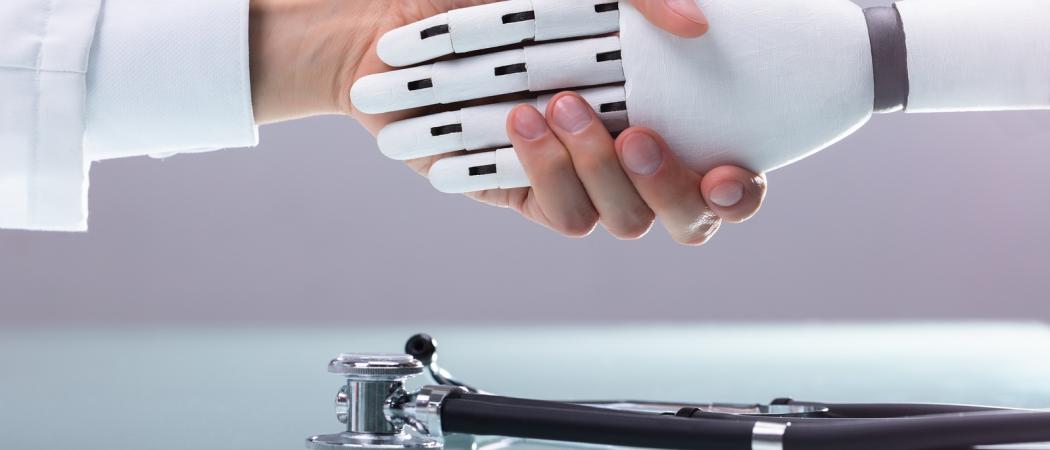
When Piotr Krajewski’s nephew was diagnosed with brain cancer, it was the beginning of a raw crash course in the healthcare system. “I got to see the problems up close,” said Krajewski, at the time manager of an IT company in Wroclaw. “It was very hard to find the specialist we needed because my nephew didn’t live in the capital.”
Surgery performed in a local hospital was a success, but a month long wait for results was agonising. “It got me thinking that maybe we can help somehow to do all this better, more efficiently and a little bit faster,” Krajewski said.
This medical odyssey was the inspiration for Cancercenter.ai, a company Krejewski co-founded in 2017, to develop deep learning image recognition technology for diagnosing and staging cancers and planning treatment.
The company claims to have developed algorithms that can interpret medical images from microscopes, magnetic resonance imaging and positron emission tomography scans, faster and better than humans. The aim is to act as an aid to speed up diagnosis, leading to faster referrals and reducing anxiety for patients
Hospitals are overstretched and need help diagnosing complex and rare conditions, Krajewski said. “The software can be a second opinion – it’s not easy for doctors to keep everything in their heads.”
However, for now in Poland, as elsewhere, the promise of artificial intelligence (AI) in healthcare is unfolding in small steps, rather than giant leaps.
Old habits take time to die, and Krajewski is discovering how hard it is to convince physicians to change how they make decisions.
“The pathologists trust their microscopes and they feel they don’t have enough time to learn how to work with new technology. In many cases, pathologists aren’t even working with electronic images yet,” said Krajewski.
The fuel for initiatives like Krajewski’s is data on which to build and train algorithms. But getting access to patient information in a sector that still relies on hand scribbled notes, is rarely straightforward for companies. Hospitals are worried about liability and feel compelled to keep patient data out of reach. If researchers or companies get permission to use data, it needs to be anonymised.
Data sets from Europe are relatively small, both because the average hospital is smaller and because regulations make it difficult to pool data from multiple facilities.
Without standardised care, or access to large enough databases, there is a limit to how good AI software can become, said Sun Yipeng, director of the European HQ of Beijing’s Infervision Technology. The Chinese company opened an office in Germany last year and has installed an incidental lung cancer finding system at the University Medical Centre in Mainz, where the aim is to screen at-risk people, including smokers, in an attempt to detect tumours at an early stage, when they are more likely to respond to therapy.
Although there is good evidence that picking up lung cancer early significantly improves outcomes, the high cost and a shortage of radiologists previously meant systematic screening was untenable.
In Spain, Infervision is working with Sant Joan de Deu Barcelona Children’s Hospital to apply its AI system to improve diagnoses of childhood respiratory diseases. There are few paediatric radiology consultants, and this could help to fill that gap.
Sun sees potential for his company’s tools to make up for a shortage of radiologists across Europe as whole. “Without specialists, general practitioners with limited time on their hands, because they have family clinics to run, are the ones who have to read the X-rays,” said Sun.
AI can “relieve doctors from repetitive, time-consuming tasks which drain their attention. The technology doesn’t have to out-perform GPs, it just has to deliver on a similar level,” Sun said.
Although there is interest in Europe for Infervision’s software, he finds European health systems overall to be less open to new AI technology than counterparts in China and the US. “You have fewer early adopters here,” he said.
Rigorous testing
Engendering trust in machine learning is gradual, rather than explosive – no one is going to risk applying these systems without rigorous testing.
However, a number of separate products that have received marketing approval from the US Food and Drug Administration underline the significant potential. For example, California-based Idx has developed an autonomous system for screening for diabetic retinopathy, without the need for a clinician to assess the image.
The FDA also approved Viz.AI’s Contact system for analysing computed tomography scans to detect stroke. The benefit seen in the clinical trials is remarkable, with Contact sending out an alert to a specialist vascular surgeon to say a patient had a clot in a large blood vessel an average of 52 minutes sooner than a first line medic conducting a standard review of the images.
Another example is diabetes, where algorithms integrated in continuous glucose monitors can predict high or low glucose events. Medtronics plc’s Guardian Connect CGM, approved by FDA in 2018, can notify users of potential hypo- or hyperglyacemic events up to 60 minutes before they are likely to occur.
The number of applications is growing fast, with big organisations including Google, Philips and IBM developing and testing systems that apply AI to health data. For example, Google has announced an add-on for light microscopes that is intended to make it faster, easier and less expensive to apply deep learning analytics to pathology. It will allow pathologists to view a real-time, AI analysis of a microscope image, superimposed upon the actual image of a pathology slide.
Countries looking to boost their AI industries are taking steps to grant easier access to medical data. French president Emmanuel Macron has pledged to make data from France’s universal health care system available for AI research.
The UK is setting up five specialist centres with £50 million of government funding, to bring AI tools into mainstream healthcare. One of these, the London Medical Imaging and Artificial Intelligence Centre for Value-Based Healthcare, led by King’s College London and based at St Thomas’ Hospital, opened in March. In addition to King’s College, St Mary’s University, Imperial College London and four NHS hospital trusts will co-locate researchers and clinicians at the centre. The industry partners IBM, Siemens Healthineers, Nvidia, GlaxoSmithKline and nine SMEs have matched the government funding.
The overall brief is to transform treatment pathways across 12 different medical specialties by using advanced imaging and AI to speed up diagnosis and triage, improving patients’ experience and clinical outcomes.
The companies involved in the project will get access to patient data to help with this. Although the exact number of records available to hone AI products has not been disclosed, just one of the clinical partners, Guys’ and St Thomas’ NHS Hospital Trust, holds a repository of 5.5 million medical images.
Knocking down barriers
If access to patient data on which to train machine learning systems is one hurdle, so too is the divide between specialties. Among those taking it upon themselves to knock down the barriers between physicians and data scientists is Leo Celi, researcher at MIT and a physician at Beth Israel Deaconess Medical Center, who organises global healthcare hackathons, or datathons, to bring the two together for freewheeling brainstorm sessions.
“Doctors might be frustrated by some of the limitations of the tools at their disposal, but they often lack the methodological background required to do something about it,” Celi said.
His monthly datathon sessions have had practical outputs. Researchers were able to tap MIT’s Laboratory for Computational Physiology open-access MIMIC database, which holds de-identified data from 40,000 intensive care patients, to develop an AI model that is better than clinicians at deciding how to treat sepsis. The results were published in Nature Medicine last autumn, and the next step is clinical trials at two hospitals in the UK.
“Computers can become a partner of physicians, a second pair of eyes, a way to augment decisions,” said Celi. Such help is essential during night shifts, he said. “The way we make decisions at 2am are going to be different to the decisions we make during the day.”
But the quest to train and validate AI systems is not helped by the EU rules on data protection. “It’s always a conundrum when you want to access data, but it’s especially hard with GDPR (General Data Protection Regulation). It has made every organisation paranoid about data sharing, and is a setback. I think we’ve swung the pendulum too far to one side and people are now discussing whether we should go back to a middle ground,” said Celi.
Fear of data misuse
Others see GDPR-style data laws as the way of the future. A recent scandal in Sweden, where the audio recordings of 2.7 million calls to a national healthcare hotline were left exposed online, underlines the need for more precautions, said Silas Olsson, director of HealthAccess Sweden, a consultancy.
“These chats went onto an unsecured server that was open for a seriously long time,” Olsson said. The calls included information about patients’ diseases and medical history. In some cases people had given their social security numbers.
As in other EU countries, Sweden is slowly edging its health sector towards the use of AI applications. “In my view, it should move with extreme caution,” said Olsson. If there are any more slips ups, “The systems will lose trust and people will hesitate to use them,” he said.
Jean-Pierre Hubaux, professor of computer communications and applications at the Swiss Federal Institute of Technology in Lausanne agrees strict rules are needed because medical data breaches “are disastrous” and “undermine the trust people have in the authorities.” Working with Lausanne University Hospital, Hubaux has developed MedCo, a system using strong encryption and the blockchain electronic audit trail of when and by whom information is accessed, to make it possible to securely share large amounts of sensitive patient data across different institutions.
For Sun, GDPR is “restrictive, but very clear on what you can and cannot do”. China’s somewhat fuzzier privacy regulation, and the country’s larger patient population, means companies like Infervision, which has amassed more than a million scans from Chinese partner hospitals, come onto the European market with an advantage.
The complexity of handling patient data to bring AI into use in healthcare, “means we’re on a long journey”, says Krajewski. “The market is not really ready everywhere for AI,” he said. “We’re on a hard, risky path, but what we’re doing is really needed.”