Ever-evolving AI systems challenge traditional notions of liability and control, a meeting of Science|Business Data Rules group hears
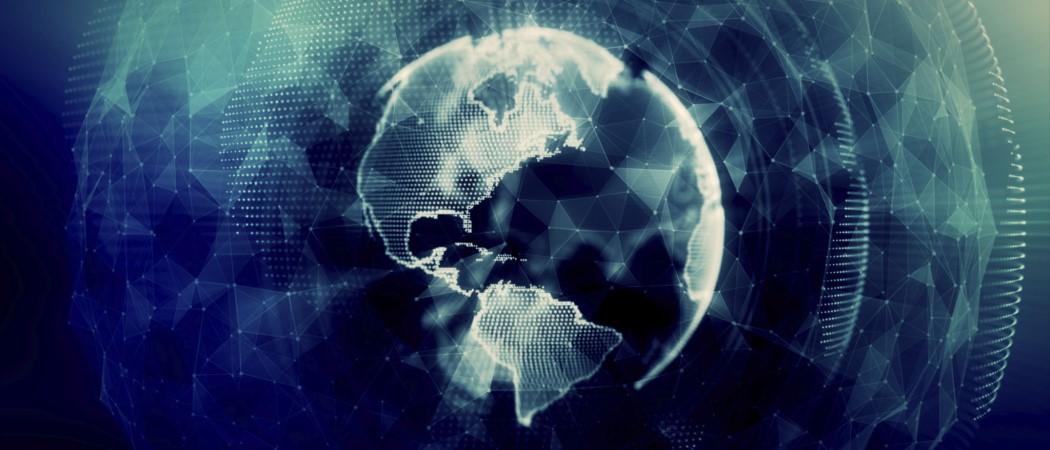
One of the greatest strengths of artificial intelligence (AI) – its ability to learn and adapt over time – could also be its Achilles’ heel.
When a product or service can learn and evolve through experience and interactions with human beings, it can be hard to pinpoint who is responsible when something goes wrong. How to allow for this dynamism and, at the same time, build trust in artificial intelligence was one of the key topics of debate in a Science|Business webinar entitled: AI: Who is Liable? - the latest in a series produced by Science|Business Data Rules group.
“You see association effects where man and machine work together in a specific context, but it is unclear what the machine has learned from man, and what man has learned from the machine,’ noted Evert Stamhuis, senior fellow at the Jean Monnet Centre of Excellence Digital Governance, Erasmus University Rotterdam.
With machine learning systems, neither the service provider nor the user may have the level of control assumed by the liability principles enshrined in law, he explained. In high risk sectors, such as healthcare, the strict liability regime proposed by the European Parliament “will have a chilling effect on innovations, so that the actual operators or users will shy away from the technology,” Stamhuis added.
This kind of approach to liability could drive AI developers to make their solutions more predictable and more explainable. But “that will lead for a time to stifling the developmental opportunities and potential of unsupervised learning systems,” Stamhuis warned.
That could mean unsupervised learning systems, in which the software figures out how to solve a problem using large volumes of unlabelled data, are abandoned in favour of supervised learning systems in which systems are trained with annotated data about appropriate outcomes or actions. AlphaGo, which has defeated the best human players of the complex game of Go, is one of the best known examples of an unsupervised learning system. After being assigned an objective by its human programmers, AlphaGo figured out how to win at Go by playing millions of games against itself.
But concerns about the social, cultural, political and economic effects of such unsupervised systems have led to a major shift towards the development of supervised learning systems in East Asia, Jack Qiu, professor & research director, Department of Communications & New Media, National University of Singapore, told the webinar.
Qiu said the techniques used by unsupervised learning systems to optimise food deliveries, for example, have prompted allegations of worker exploitation and suicides. He argued that AI systems that impact people’s lives need to be carefully controlled and should be governed democratically by the individuals that they affect. “The rules are really up to the working people whose livelihoods are at stake,” he said. It is “better to depend on them rather than on the big corporations or bureaucracy,” he added.
In a similar vein, Paul Nemitz, principal advisor, DG Justice and Consumers, European Commission, argued that the future vibrancy of democracy will depend on ensuring that no single entity assumes too much technical power and maintaining a healthy degree of scepticism among citizens. “We should not teach [children] trust in technology,” he said. “We should teach a critical attitude and we should teach language in order to participate in the great debates of democracy. I think that's the challenge of the time.”
Can a machine explain itself?
One of the ways to democratise AI would be to make its decision-making processes transparent and explainable. This is the subject of several major research programmes and is one of the objectives of the US$2 billion AI Next campaign being run by DARPA in the US. Christopher Hankin, professor of computer science and co-director of the Institute for Security Science and Technology, Imperial College London, warned that this will be difficult to achieve, even with supervised systems, which he said can be as inexplicable as unsupervised systems. “In supervised learning, often, particularly if you're using neural net technology, there are many different layers to the neural network and it's very difficult to extract why a particular decision might be being suggested,” he said. Hankin was speaking partly on behalf of the Association of Computing Machinery.
The speakers also debated whether the explicit consent mechanisms in the General Data Protection Regulation (GDPR) can be adapted to ensure consumers understand how AI systems might use their personal data. “If you buy software today […] the terms will include a blanket disclaimer of liabilities attached to the use of that software,” Hankin noted. “Maybe that's something we need to seriously revisit in the context of AI-based systems and automated decision making systems.”
One approach is to provide users of AI systems with a clear and detailed explanation of the risks involved, akin to the leaflet that describes the potential side effects of medicines. But that may be easier said that done. In the case of a neural network-based system, once it has been configured, “it's very difficult for anyone to really unpick why the algorithm works in the way that it actually does at any particular point in time,” Hankin said, noting this makes it very difficult to fulfil the GDPR’s requirement to provide “meaningful information about the logic involved.”
In some fields, experts will act as intermediaries. When an AI system is used to guide healthcare, a physician will often combine the information provided by the AI system with their own medical expertise and provide appropriate explanations to the patient in order to obtain the patient’s informed consent. Realising the full potential of AI in healthcare will depend on striking the right liability balance between the three actors in the triangular relationship between the AI system, the physician and the patient, Yiannos Tolias, legal officer at DG SANTE, European Commission, told the webinar. He suggested that liability frameworks should be designed to encourage collaboration between these three actors.
Although he favours making AI systems as explainable as possible, Tolias cautioned that transparency can create a false sense of security. “In recent research, it has been found …sometimes the physician over trusts the explanation and follows the explanation, even if the explanation is not really correct.” he said. “So we can see that explainability, which we thought at some point to be a panacea,” could present new challenges that will also need to be addressed.
Expert checks and balances
Another potential solution is to require AI systems to be subject to audits by third party experts, just as plans for new buildings are inspected by independent surveyors. “It is completely appropriate for many tasks to have that kind of inspection as part of the process,” Fred Popowich, professor of computing science and scientific director of Simon Fraser University’s Big Data Initiative, told the webinar from Canada,.
As the dynamic nature of AI systems means a one-off audit may not be sufficient, Popowich also agued that human beings may need to regularly ensure that the data that the AI system is learning from is both up-to-date and representative. “We've seen advances for identifying and mitigating bias in AI algorithms and there's a lot of exciting work still to be done,” he added.
Popowich also cautioned against becoming too fixated on the concept of reproducibility – ensuring an AI system will reproduce an earlier decision. “Humans won't necessarily make the same decision at different points in time because something has changed,” he added. “It's not just about data-driven approaches. It's about information-driven approaches, knowledge-driven approaches and maybe wisdom-driven approaches.”
Balancing the risks and rewards
More broadly, the potential risks of using AI systems clearly need to be balanced with the potential benefits. In healthcare and other fields where human resources can be highly stretched, placing too many restrictions and safeguards on AI systems could be counterproductive. “One thing to maybe have in mind […] is whether the end result is at least as good as, and as reliable as, the human made equivalent,” noted Elizabeth Crossick, head of government affairs – EU at RELX, which provides analytics and decision tools. She suggested this criteria could be an important baseline.
For RELX and other global companies, the international harmonisation of the rules around AI is also important: a patchwork of different data and AI rules in different countries could make it harder to develop and apply this technology effectively. Crossick called on policymakers to provide “more positive support for AI getting to market,” adding “the EU won't create open strategic autonomy, unless it commits to being an early adopter and broad user of the best technologies that are on offer and that will be created.”
The EU is in the midst of developing new AI legislation, designed to provide a consistent regulatory regime and level playing field across the EU. Paul Nemitz of the European Commission called on stakeholders to encourage their national governments to pursue the harmonisation of rules across borders, noting that cross-border scientific research has been impacted by gaps in the GDPR.
But Nemitz stressed that the GDPR provides a solid and sustainable foundation for the development of safe and trusted AI systems. “It was definitely the intention of the lawmaker to make a technology-neutral law, that's why you don't find the buzz words of the day in the text, and this also means that we have to interpret the text ever new with new technologies” he said. “There's a very simple rule in EU law, which is if the secondary law is unclear, look to the primary law and we have a very good provision on data protection rights in the charter of fundamental rights.”