Researchers say AI models are underused because the data they need is kept hidden for commercial reasons
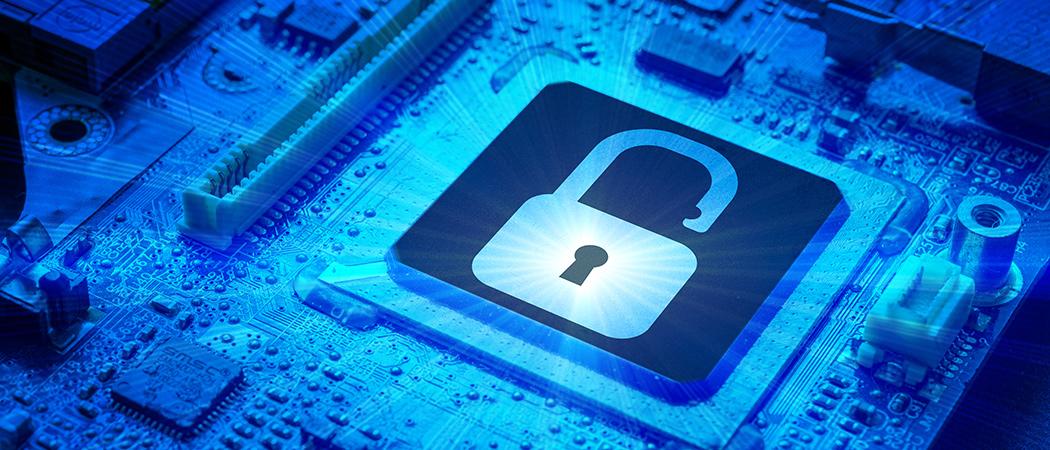
The discovery of new drugs is being held back because pharmaceutical firms are not sharing their data, limiting the potentially revolutionary impact of artificial intelligence on the field, according to AI experts.
AI systems can sift through millions of molecules to search for candidate drugs, opening the door to a much faster path to new treatments.
Last year, for example, a team at the Massachusetts Institute of Technology reported discovering a new antibiotic compound using a computer model that can screen more than 100 million compounds in a matter of days.
But such breakthroughs are being hampered by a lack of data sharing by private companies, stymying efforts to use powerful AI models to improve healthcare, said Yoshua Bengio, an AI pioneer at the University of Montreal and one of the leaders of an OECD-backed investigation into the issue.
“The lack of open datasets is a failure of the principle of profit maximization by individual actors,” he said.
Releasing datasets “hurts their competitiveness, even though it would help the overall market to progress faster to technological solutions,” Bengio said.
Last month, a report by Bengio and other AI experts warned that the present system is “suboptimal for AI research, and this threatens to limit the positive impact of AI.”
“The field requires a shift towards open data and open science in order to feed the most powerful, data-hungry AI algorithms,” says Artificial Intelligence for Public Domain Drug Discovery, presented at the annual conference of the Global Partnership on Artificial Intelligence (GPAI), an initiative launched in 2020 under French and Canadian leadership.
In the academic community, data sharing has taken off, and is now mandatory under most government funded grants, said Bengio. Researchers are rewarded through downstream citations if they allow others to use their data.
But the incentives for the private sector are still to keep data closed. Companies need to be encouraged to share their data, “by force of contract and financial rewards for doing the right things”, Bengio said. The GPAI report also calls for government intervention to “strongly encourage” data-sharing.
Weight in gold
AI-assisted discovery has made huge strides in areas like speech recognition and computer vision where big datasets are public, Bengio noted. Decades of sharing protein crystal structures by scientists had allowed Google subsidiary DeepMind to train its AlphaFold algorithm to predict a protein’s structure from the amino acid sequence, creating a “real revolution in biology”, he said.
Now Bengio and colleagues want similar openness when it comes to drug discovery.
“High quality data is worth its weight in gold to us,” said Elliot Layne, a researcher at McGill University, presenting the report in Paris last month. “But what we see here inside this industry is frankly there’s a lack of high-quality, open source datasets.”
Keeping datasets private can offer companies “a significant competitive advantage,” he said, creating a “barrier to innovation on problems where we can’t afford to take our time.”
Private sector caginess is not the only thing stopping AI systems discovering new drugs, the GPAI report says. There’s a lack of coordination between different domains, with pharmaceutical researchers and AI experts not joined up enough.
What’s more, applying AI to datasets requires massive amounts of computing power. “The scale of compute needed for state-of-the-art algorithms is becoming prohibitive for many smaller players, such as most academic labs or early-stage startups, or even larger companies,” it says.
Along with AI access to proprietary chemical libraries, academic researchers need access to clinical trial data and data on how a drug’s efficacy and side effect profile is impacted by a patient’s genetic makeup, it adds. Finding these kinds of datasets is often much harder than accessing the chemical data needed for early stage discovery, the report says.
Market failure
The wider picture, say Bengio and other experts, is that the drug discovery ecosystem is misfiring for a range of reasons, not just because of a lack of datasets for AI to work upon.
“Some innovations are crucial for society…but are not happening because of a market failure,” he said.
Despite the much-praised rapid development of vaccines against Covid-19, it typically takes 10-12 years to bring a new drug to the market.
Research and development on new antibiotics that are needed to address the rise of antibiotic resistance has “almost completely ground to a halt”, the report says. Amongst other issues, this is because new antibiotics must be used sparingly to preserve their effectiveness, limiting the commercial return on developing them.
“This is absolutely a crisis that calls for coordinated global action, and it’s critical that we use every tool at our disposal to address this, including the sky-high impact that AI could have,” said Layne.
“Unfortunately what we see here today is that this work so far is just not being done, and in fact many large pharmaceutical companies are decreasing the amount they invest into researching novel antibiotics, or in fact just dropping the work completely,” he said.
In fact, since the alarm on antimicrobial resistance was sounded in 2014 by the UK government sponsored O’Neill report, a number of global initiatives have taken shape to address this crisis. Most recently, in July 2020, 23 pharma companies set up the AMR Action fund, raising $1 billion for the clinical development of antibiotic drugs addressing the most resistant bacteria. After shunning antibiotics over the previous decade, the companies said they will strengthen and accelerate their development.