Extracting information quickly from quantum states is necessary for future quantum processors and super-sensitive detectors in existing technologies.
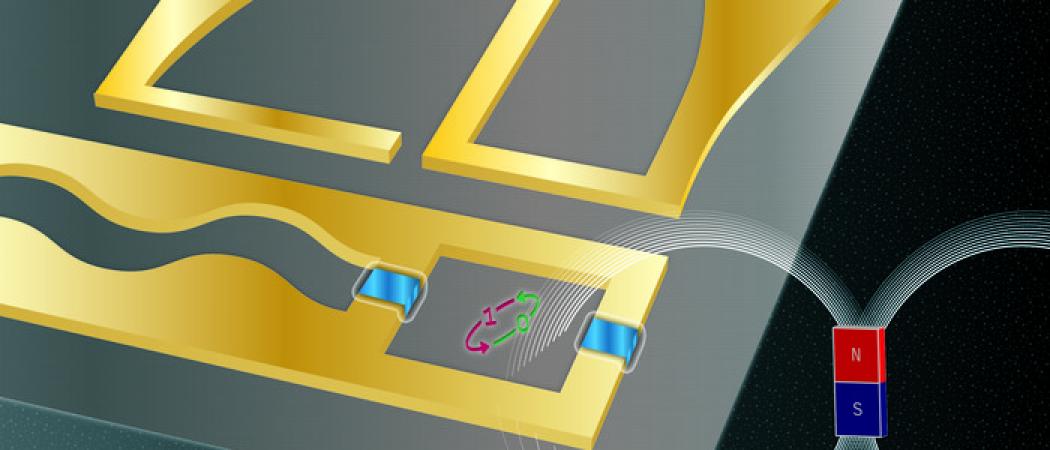
An artificial atom realized from superconducting strips of aluminum on a silicon chip can be employed for the detection of magnetic fields. Image: Babi Brasileiro / Aalto University
There are limits to how accurately you can measure things. Think of an X-ray image: it is likely quite blurry and something only an expert physician can interpret properly. The contrast between different tissues is rather poor but could be improved by longer exposure times, higher intensity, or by taking several images and overlapping them. But there are considerable limitations: humans can safely be exposed to only so much radiation, and imaging takes time and resources.
A well-established rule of thumb is the so-called standard quantum limit: the precision of the measurement scales inversely with the square root of available resources. In other words, the more resources – time, radiation power, number of images, etc. – you throw in, the more accurate your measurement will be. This will, however, only get you so far: extreme precision also means using excessive resources.
A team of researchers from Aalto University, ETH Zurich, and MIPT and Landau Institute in Moscow have pushed the envelope and came up with a way to measure magnetic fields using a quantum system. They demonstrate a new method that combines quantum phenomena and machine learning to realise a magnetometer with accuracy beyond the standard quantum limit.
The detection of magnetic fields is important in a variety of fields, from geological prospecting to imaging brain activity. The researchers believe that their work is a first step towards of using quantum-enhanced methods for sensor technology.
‘We wanted to design a highly efficient but minimally invasive measurement technique. Imagine, for example, extremely sensitive samples: we have to either use as low intensities as possible to observe the samples or push the measurement time to a minimum,’ explains Sorin Paraoanu, leader of the Kvantti research group at Aalto University.
Their paper, published in the prestigious journal npj Quantum Information shows how to improve the accuracy of magnetic field measurements by exploiting the coherence of a superconducting artificial atom, a qubit. It is a tiny device made of overlapping strips of aluminium evaporated on a silicon chip – a technology similar to the one used to fabricate the processors of mobile phones and computers.
When the device is cooled to a very low temperature, magic happens: the electrical current flows in it without any resistance and starts to display quantum mechanical properties similar to those of real atoms. When irradiated with a microwave pulse – not unlike the ones in household microwave ovens – the state of the artificial atom changes. It turns out that this change depends on the external magnetic field applied: measure the atom and you will figure out the magnetic field.
But to surpass the standard quantum limit, yet another trick had to be performed using a technique similar to a widely-applied branch of machine learning, pattern recognition.
‘We use an adaptive technique: first, we perform a measurement, and then, depending on the result, we let our pattern recognition algorithm decide how to change a control parameter in the next step in order to achieve the fastest estimation of the magnetic field,’ explains Andrey Lebedev, corresponding author from ETH Zurich, now at MIPT in Moscow.
‘This is a nice example of quantum technology at work: by combining a quantum phenomenon with a measurement technique based on supervised machine learning, we can enhance the sensitivity of magnetic field detectors to a realm that clearly breaks the standard quantum limit,’ Lebedev says.
Aalto researchers acknowledge the project QMETRO of the Centre for Quantum Engineering and the Academy of Finland Centre of Excellence in Quantum Technologies. The research made use of the national OtaNano research infrastructure.
Research article: S. Danilin, A.V. Lebedev, A. Vepsäläinen, G.B. Lesovik, G. Blatter, and G.S. Paraoanu, Quantum-enhanced magnetometry by phase estimation algorithms with a single artificial atom. npj Quantum Information (2018) 4: 29.
https://www.nature.com/articles/s41534-018-0078-y
doi:10.1038/s41534-018-0078-y