Experts debate how best to harness the vast amounts of data being generated every second
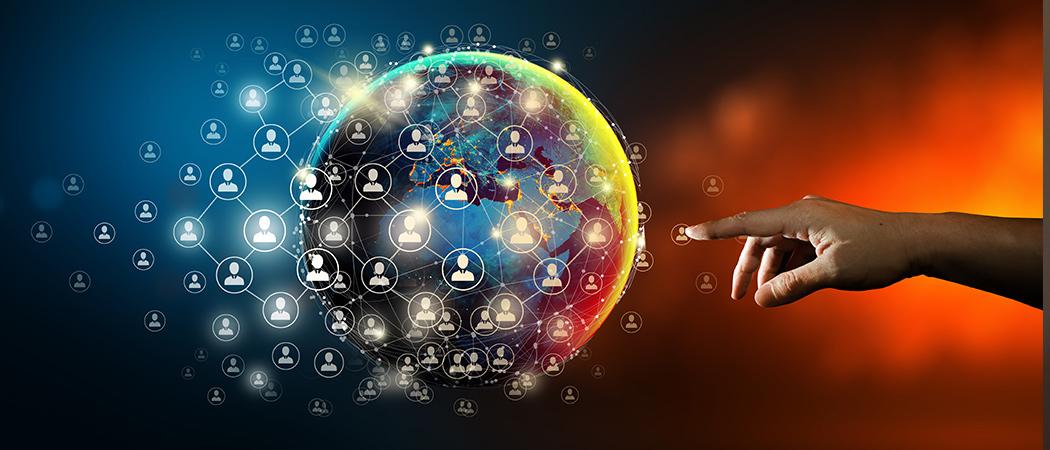
Unlike many valuable resources, real-time data is both abundant and growing rapidly. But it also needs to be handled with great care.
That was one of the key takeaways from an online workshop produced by Science|Business’ Data Rules group, which explored what the rapid growth in real-time data means for artificial intelligence (AI). Real-time data is increasingly feeding machine learning systems that then adjust the algorithms they use to make decisions, such as which news item to display on your screen or which product to recommend.
“With AI, especially, you want to make sure that the data that you have is consistent, replicable and also valid,” noted Chris Atherton, senior research engagement officer at GÉANT, who described how his organisation transmits data captured by the European Space Agency’s satellites to researchers across the world. He explained that the images of earth taken by satellites are initially processed at three levels to correct for the atmospheric conditions at the time, the angle of the viewpoint and other variables, before being made more widely available for researchers and users to process further. The satellite data is also “validated against ground-based sources…in-situ data to make sure that it is actually giving you a reliable reading,” Atherton added.
Depending on the orbit of the satellites and the equipment involved, the processing can take a few hours or a few days before it is made available to the wider public. One way to speed things up post publication is to place the pre-processed data into so-called data cubes, Atherton noted, which can then be integrated with AI systems. “You can send queries to the data cube itself rather than having to download the data directly to your own location to process it on your machine,” he explained.
Keeping up with reality
Some data, such as that relating to extreme weather or short-term pollution, can be very time-sensitive: people and organisations may need to be alerted to fast changing environmental conditions that could endanger them or their property.
AI can potentially compensate for a lack of readily accessible real-time data by making near-term forecasts based on the latest data available. This is the approach taken by BreezoMeter, a start-up that provides both consumers and major companies with real-time information and forecasts for air quality, pollen counts and wild fires. “We have used machine learning in algorithms or other dispersion models to take the near real time [data] and convert it into real time,” Ran Korber, CEO of BreezoMeter, told the webinar.
BreezoMeter provides localised information at a resolution of up to five metres in 100 countries, including places where public agencies don’t monitor air quality. “About 120 million Americans live in areas when the U.S. EPA (Environmental Protection Agency) doesn't have any measurements about several pollutants,” Korber said. In such cases, BreezoMeter draws on data on other factors, such as traffic levels, to forecast the volume of pollutants in a specific area. It feeds data from 10 million different sources into models that employ more than “40 unique proprietary algorithms” to forecast concentrations of more than 30 different pollutants, Korber said.
Crowdsourcing data in 3D
BreezoMeter also collects data from its users. Such crowdsourcing is playing an increasingly important role in the data economy and could ultimately enable scientists to build very detailed three-dimensional dynamic models of reality. Manolis Savva, assistant professor at the School of Computing Science, Simon Fraser University in Canada, envisions a “world where creating and personalising and sharing 3D content that is meaningful to us will become as easy as just taking a photo with a smartphone and then sharing it with other people.”
In future, we can expect to have access to a “real-time highly contextualized high fidelity data” feed about our lives, he added. “We will have more and more information that captures our everyday context.” Savva noted that some smartphones now contain Lidar (light detection and ranging) sensors that can map out their surroundings.
Society needs to consider how such data could be “accumulated or leveraged by particular organisations,” he added, noting there are important questions to address, such as: “What frameworks should we put in place to maximise the utility of all of this data that we will be able to collect to everyone?” He also stressed the growing importance of preserving the “metadata that goes along with the data”, so there is a clear record of its source and what it represents.
Humans can be unreliable data sources
If data collected by scientific instruments needs to be cleaned and processed before it is employed by AI systems, data generated by human beings comes with an even bigger health warning. Gui Liberali, professor of digital marketing, Erasmus University Rotterdam, noted that when you attempt to monitor/observe what people online are thinking, you may also affect what they are thinking, as well as invading their privacy. Aware that they are being monitored, some people will deliberately try to out-smart an AI system. Liberali pointed out that online buyers of airline tickets might “actually go into incognito mode or they open three browsers to manipulate what firms are offering to them to get a better perception.”
If fickle and offensive opinions are expressed and disseminated through machine learning systems that could have an undesirable impact on society. “Should we just let people say what they want or not,” no matter what? Liberali asked. “Where do we draw the line?” He noted that left unchecked the algorithms managing social media could destroy “economic and social and political structures in society, as we saw a little bit a few years ago.” Citing a book (The Hype Machine) by Sinan Aral, Liberali pointed to four levers that society can use to create checks and balances on social media: business model restrictions, social norms, laws and algorithms that can check the algorithms generating data. Rather than looking at content during a crisis, the idea is to create code and incentive systems that can prevent crises.
In cases where machine learning systems evolve beyond a certain threshold, they could be configured to shut themselves down. “I think there definitely needs to be, let’s say, a panic button,” a bit like circuit breakers in stock markets, Liberali said.
Unfortunately, ensuring that both the algorithms and the data they process are fair and robust isn’t sufficient to prevent misuse, cautioned Anjali Mazumder, theme lead on AI & justice & human rights at The Alan Turing Institute in the UK. “You could have a tool which it's been determined it is robust, it is fair, but its use is another case,” she noted, pointing out that the use of real-time facial recognition technology in some situations could be very concerning.
At the same time, data doesn’t always flow to where it is needed most. “We saw in the UK during the pandemic, and globally, of course, that depending on the infrastructure, hospitals weren't connected,” Mazumder noted. “Various agencies within the health sector were not connected enough to be able to access, and therefore, support our government in their response in real time, or even in less real time, for that matter.”
She called for more investment in infrastructure and incentives to encourage the sharing of data both to enhance innovation and improve policymaking. As things stand, researchers face the challenge of having to “constantly keep asking for the same data,” Mazumder explained.
Indeed, the need for a well-informed response to COVID-19 underlined the importance of being able to rapidly share fresh data during an emergency. Adina Braha-Honciuc, sustainability policy manager at Microsoft, suggested climate data could be classified as emergency data. Such a step would “accelerate progress in that space and make sure that the collaborative analysis of these data sets are made… more available and easier to address,” she contended.
Trusted data markets with neutral intermediaries
To facilitate faster, safer and smoother data sharing, the European Commission is developing both a regulatory framework, and common data spaces, which will act as marketplaces for data. Antonio Biason, legal & policy officer in DG CNECT, said intermediaries will play a key role in matching sources of supply and demand. The new EU Data Governance Act will require these “data intermediaries” to be entirely neutral. “They would create the link between the data holder and the data re-user,” Biason explained. “That neutrality is what ensures difference between the European model of dealing with data sharing and other models around the world.”
One measure of the success of the data spaces will be the extent to which they accelerate the sharing of data across sectors, Biason added. “The data would be formatted in such a way that it would be truly interoperable…so data that would be collected, for instance, for satellite purposes could also be used for the mobility sector, as well as for the energy sector.” The end result, he added, could be a “true genuine single market for data which will inevitably lead to innovation beyond our wildest dreams.”
Entities outside the EU will be able to participate in the data spaces “as long as they comply with the European rules and values,” such as the preservation of security and privacy, Biason added. “The data economy is not something that can be just closed off. …a small circle of actors will never be as strong as something that indeed includes a vast or an open number of participants.”