Science|Business workshop explores how Europe should respond to the rapid advances in the performance and utility of artificial intelligence
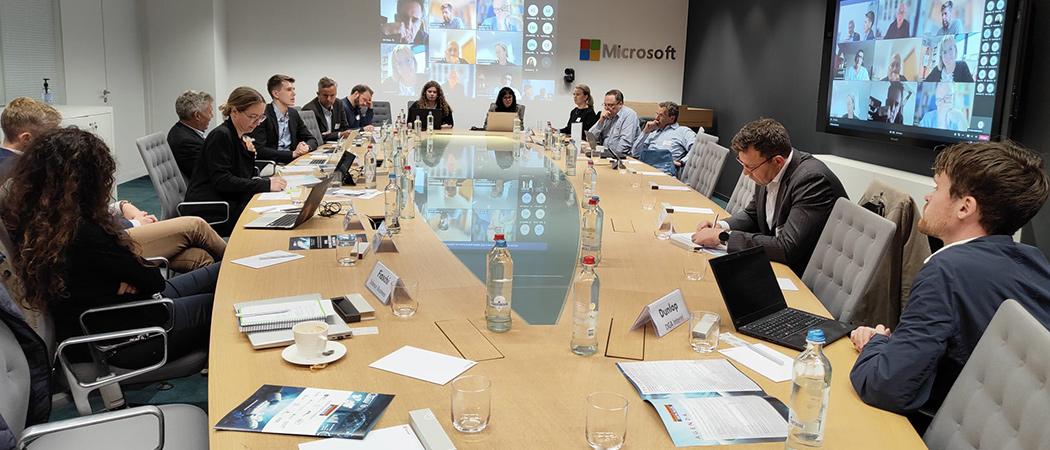
Hosted by Microsoft, the workshop brought together experts online and in-person to debate how super computers are shaping artificial intelligence. Photo: Science|Business
Artificial intelligence is developing in leaps and bounds as new systems harness the capabilities of supercomputers and dedicated semiconductors. But the ongoing acceleration in AI performance and utility raises a number of challenges for policymakers grappling with how to ensure their countries remain competitive and how to regulate this dynamic and far-reaching technology.
Those were two of the messages delivered by the experts participating in a Science|Business Data Rules workshop on the Impact of Advanced Computing on AI. In particular, the meeting explored the ramifications of the recent step change in the size of AI models – the neural networks that learn how to perform specific tasks by detecting patterns in training data.
“In the last five years, the largest scale models have increased 300,000 fold in size,” Andreas Ebert, national and industry technology officer of Microsoft told the workshop. “Currently AI models are doubling every three to four months. And even this speed is accelerating.”
But these advances come at a price. Ebert highlighted how the cost of a single training run can now be as much as €50 million. Microsoft and other major tech companies are increasingly investing hundreds of millions of dollars in building the supercomputers required to run massive AI models. At the same time, tech companies are designing semiconductors specifically to support AI, while also using AI to design new hardware.
As HPC (high performance computing) and AI advance in tandem, a virtuous circle is developing, noted Anne Elster, professor of computer science and founder of the Norwegian University of Science and Technology’s HPC-Lab. “You have HPC for AI and you now have AI for HPC. They now impact each other.”
How risky is general purpose AI?
Many neural networks are now so capable that they are referred to as general purpose models in the sense that they can be used to build a very wide range of applications from speech transcription to facial recognition. As these applications vary from low risk to high risk, general purpose systems are difficult to regulate.
The current draft of the EU’s AI Act may be starting from the wrong point because it fails to take into account how AI models are developed and how they are integrated into systems, and what kind of decisions will be made when they are deployed. Highlighting the importance of the allocation of responsibilities, Cornelia Kutterer, senior director, EU government affairs, AI, privacy and digital policies at Microsoft, noted that the user of the AI system, who is closest to the high-risk scenario in which the system is deployed, makes important decisions that can have an impact on the system's performance. This is not appropriately taken into account in the draft of the AI Act, she contended.
Just as the user must be made aware of the capabilities and limitations of the AI system they deploy, the AI system provider will need to ensure that software components providers, pre-trained AI models or general purpose AI deliver the relevant information to them, Kutterer added, noting that the AI Act instructs these providers to collaborate, and this will ultimately be reinforced by contracts in the AI stack. The AI Act should continue to focus on AI systems used in high-risk scenarios, she stressed.
The challenge for regulators is that foundational models, some of which are open source, could be applied in multiple different ways for both good and nefarious purposes. While they can be adapted to write software code, predict molecular sequences and perform other valuable tasks, there is also the risk that they are used to create fake videos or images and propagate extremist content. There is also the danger that any biases in the data used to train general purpose models will be carried through to many different AI applications.
As these general purpose models are highly malleable components, rather than end products, the line between the provider and the user can become very blurred. “Where do you place the responsibilities, and what responsibilities do you place where?” asked Kris Shrishak, technology fellow at the Irish Council for Civil Liberties. Advocating that the upstream providers of general purpose models should be responsible for their performance, he noted that some of the proposed amendments to the EU AI Act are trying to address these questions. “We're moving in a direction where we might have something reasonable.”
Flagging that some general purpose models exhibit unexpected capabilities, such as being able to write code, Nicolas Moës, director of European AI governance at The Future Society, said there is not yet a clear way forward on how to address this issue without hindering innovation. “We are really looking at a situation where the regulators do not know what to do, and the developers themselves, do not know,” he contended, noting that these foundational models could play a major role in developing the next generation of machine learning systems.
Indeed, the growing automation of software development could make it increasingly difficult for law enforcement agencies to unpick what happened in the event that a decision by an AI system causes harm to an individual or an organisation.
Moës proposed maximising the amount of interactions between regulators and AI developers to bolster understanding on both sides. To that end, he advocated using regulatory sandboxes, which allow for a temporary relaxation of some rules under supervision, as well as the appointment of specialist regulatory staff that can interact with AI developers. Moës suggested deploying a dedicated and well-resourced regulatory team with expertise in general purpose AI.
But equipping regulators (and enforcement agencies) with the necessary skills and resources to check foundational models could prove expensive, given the complexity and scale of the latest generation of neural networks. Fully understanding the behaviour of foundational models will require a systematic analysis of their learning processes and the data they are based on, according to Sofia Vallecorsa, a researcher with CERN. She argued that the EU should harness its HPC capabilities to help make these models explainable and transparent.
Afonso Ferreira, scientific coordinator for European affairs, CNRS Institute for Computer Sciences INS2I, stressed how difficult it is to find people who understand both technology and policy, which move at completely different speeds. He is participating in a Marie Curie initiative to train a cohort of 15 PhD students on how to become data scientists that are aware of the legal framework that can sometimes hamper their work.
Europe’s big bets on high performance computing
Aware of the increasing strategic importance of supercomputing, the European Commission is part-funding the EuroHPC Joint Undertaking, which has a budget of €7 billion. Some of that money will be used to bring new supercomputers online between 2021 and 2027. The hope is that this new capacity will help Europe become more competitive in computing in general and AI in particular.
“It really means that Europe is catching up in terms of providing compute capabilities and the performance,” Dirk Pleiter, director of the PDC Center for High Performance Computing, KTH Royal Institute of Technology said. However, he stressed that most supercomputers are not yet optimised to support AI. “There is still also some work which needs to be done …to make the systems that are accidentally good for machine learning [more] suitable for machine learning” workloads.
Pleiter also cautioned that “the systems are mainly operated… in the classical HPC way.” As each application is reviewed by a panel, it can take several months for an applicant to gain access to the supercomputing resources. He acknowledged that many people working in the AI and machine learning field would like to have much more flexible access to large scale computing resources. “There is no elasticity there,” Pleiter cautioned.
While the EuroHPC Joint Undertaking is investing in particular in hardware, Pleiter suggested there is a more urgent need in the short-term to provide small businesses and scientific research groups with the expert support they need to actually run AI models on HPC systems.
Containing computers’ expensive energy usage
Like most of Europe’s economy, the operators of supercomputers are grappling with the rising cost of the energy they use. For example, for CNRS in France, which has 35 petaflops of computing capacity available for scientific research, one of the big issues “that we are facing now is … the energy bill,” Ferreira said.
Tomasz Trzcinski, computer vision group leader, at IDEAS NCBR, suggested that the EU should take the lead in developing energy-efficient HPC and AI. He said the focus should be on “getting the most out of what we already have instead of increasing the size of the model.”
One of the reasons the cost of training models is so high is because researchers “don't think about what has been done before and recycle or reuse it. They just run another test,” he argued. “Now when you say … train the best model that you can with 100 bucks or five megawatts or whatever the constraint is… that gives a very different perspective on where we can be the forefront of the world's AI development.”
Ebert of Microsoft stressed that it is far more energy efficient to implement all the necessary computing in a single location than to shuttle data across long distances in a federated computing architecture. The development of semiconductors specifically designed for AI should also help to reduce energy costs. Ebert said it may be possible to achieve a ten to one hundred fold improvement in energy usage through dedicated chip designs.
Jean Pierre Panziera, chief technology officer, Atos HPC, AI & QC business line, described how his company is partnering with Graphcore, a UK-based semiconductor company that is trying to develop an AI computer that will be able to surpass the parametric capacity of the human brain. It intends to have built a neural network that can handle more than 100 trillion parameters within three years. “That computer will consume something around the 20 megawatts with the technology that we are foreseeing there,” Panziera said. That would mean it would consume as much power as 13,000 homes.
Revitalising computer science in Europe
Graphcore not withstanding, Europe’s semiconductor industry lags well behind its counterparts in the U.S. and East Asia. Panziera believes Europe needs to close that gap to be more competitive in the development of AI and to reduce the geopolitical risks of being dependent on other regions for key technologies. “You need to be able to master that technology,” he said. “We need to make sure that we get back to having a semiconductor industry that is up to speed, up to the level of the best in the world.”
Many of the biggest tech companies are now designing semiconductors and developing the corresponding AI software in tandem. Vallecorsa of CERN argued that this kind of integrated development will yield much better results that developing large AI models and then trying to optimise them to run on a specific kind of hardware.
Another key strategic question for Europe is how much to invest in quantum computing, which is seeking to use the principles of quantum physics to enable computers to acquire a wider range of capabilities.
Alba Cervera Lierta, a senior researcher at Barcelona Supercomputing Center, told the workshop that quantum computers are likely to be integrated into existing HPC centres so that AI models and other applications can harness both quantum and classical computing hardware. “So for instance, a quantum computer will act as an accelerator, which means that some part of the algorithms will be run in this particular machine, while other kinds will be run” on more conventional computing architectures, she explained.
Europe will have to develop its own quantum computing electronics, Lierta added. “In quantum, we make our own chips or we wouldn't have a quantum computer,” she said, noting that other regions are unlikely to share cutting edge quantum technology with Europe. Although it may be some time before the performance of quantum computing surpasses classical computing, Europe needs to continue investing substantial sums in quantum technology, according to Lierta. “If we wait, then once everything works, we will need to start up again,” she said. “And it will take 20 years to develop and these are exactly the discussions that we are suffering with semiconductors right now.”
For some forms of computing, “it has been proven that a quantum computer will be more efficient in terms of energy, like orders of magnitude,” Lierta added. Ebert echoed that view, pointing out that quantum computers are likely to work within a “fixed energy budget” regardless of how many quantum bits they need to process.
Can Europe figure out the way forward?
The complex interplay between AI and advanced computing hardware raises strategic dilemmas for Europe. While Europe has a very strong scientific base, its commercial computing base is relatively weak. Yet it is becoming increasingly difficult to delineate commercial and research IT infrastructure – both scientists and commercial AI developers require access to very advanced computing capabilities.
At the same time, the costs of using this advanced infrastructure is making it increasingly difficult for scientists to verify each other’s work or for regulators to check what commercial AI models are capable of and how they reached specific conclusions.
With computing technologies developing quickly on a number of fronts, regulators are likely to find it tough to produce prescriptive rules. As a result, they may have to rely on a principles-based approach, which is flexible enough to be applied to new computing paradigms.
Another major challenge will be getting the right balance between digital sovereignty and sustainability – running power-hungry computing resources in places where energy is generated by fossil fuels will become increasingly hard to justify, as both the financial and environmental costs escalate.
Finally, there is no clear consensus on how much control European companies and governments should try to exert over the design and supply of semiconductors. Given the need to maximise performance within a reasonable energy and financial budget, specialist chips will be an increasingly important component of AI models and the broader computing stack. Tesla, for example, is designing semiconductors optimised for the AI it is developing to enable self-driving cars.
Some large European companies could try and follow suit, but they would probably remain reliant on businesses based elsewhere to supply the actual chips. That’s because building new plants to manufacture advanced chips would be a very expensive gamble - the cost of a single state-of-the-art chip factory is now as much as €40 billion.