Researchers at Japan’s RIKEN join global hunt for a better way to forecast extreme weather events. The challenge: how to scale up expensive trial systems into routine tools for all
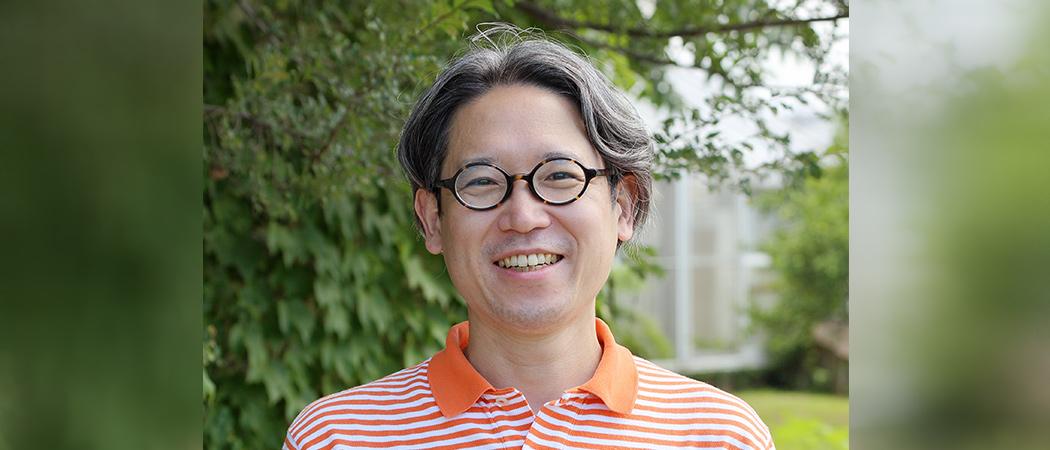
Takemasa Miyoshi, Data scientist in the Centre for Computational Science at RIKEN
It’s no exaggeration to say that some rainstorms come out of a clear blue sky. They develop so quickly, and are so local, that conventional weather forecasting systems simply don’t see them coming. This was the case in Kobe, Japan, in the summer of 2008. Clouds quickly formed; there was a heavy burst of rain; and within 10 minutes a small river running through the city had risen by more than a metre, killing five people.
“If we could predict an event like that even 30 minutes in advance, people could easily escape,” says Takemasa Miyoshi, a data scientist in the Centre for Computational Science at RIKEN, Japan’s largest comprehensive research institution.
So, he and his colleagues set out to design a system that could predict the formation of individual rain clouds much more precisely. Theirs is a work in progress: They have run a series of trials on supercomputers that succeeded in predicting heavy rains in Tokyo this summer, but there’s still a long way to go in scaling up to a live extreme-weather warning system.
Yet the attempt itself is important, and part of a global effort to improve our forecasting tools as the connection between climate change and extreme weather events becomes clearer. This month’s report on climate hazards from the European Environment Agency, for example, states that climate change due to human activities is now “undeniably responsible” for an increase in extreme weather events in Europe, and that people across the region need to be prepared.
The seriousness of this warning is apparent in the heavy rains that caused flooding in Germany and Belgium this July, resulting in more than 200 deaths and several billion euros worth of damage. Similar disasters hit other parts of the world. Also in July, extreme rainfall in China’s Henan Province resulted in flash floods that were linked to more than 300 deaths, with reported economic losses estimated at $17.7 billion. Meanwhile, persistent above-average rainfall in the first half of 2021 led to significant, long-lasting floods in the north of South America. Floods also hit parts of East Africa, with South Sudan particularly badly affected.
Needed: better data, faster computers
Weather forecasts can see some of these extreme rain events coming; the European floods were predicted days in advance. But others still take us by surprise. This can be because of deficiencies in weather models, a lack of sufficiently detailed data for them to work with, or insufficient computing power to produce a timely result. These bottlenecks are being addressed by major research initiatives, such as the World Climate Research Programme’s Grand Challenge on Weather and Climate Extremes, and the World Meteorological Organisation’s High-Impact Weather Project, part of the World Weather Research Programme.
Meanwhile the EU has funded the CAFE project (Climate Advanced Forecasting of sub-seasonal Extremes), which is training young researchers in subjects such as climate science, complex networks and data analysis, in the hope this will lead to interdisciplinary research that improves the predictability of extreme weather events. The project is already bearing fruit, for example with a study from the European Centre for Medium-range Weather Forecasts and TU Freiberg that developed a framework to better predict extreme rainfall events in Mediterranean countries.
The project carried out by Takemasa Miyoshi at RIKEN deals with more local, extreme weather events, and addresses shortcomings in the data going into the model. As such, it’s a snapshot of just one of the many problems that meteorologists are encountering as they try to improve their climate-related forecasting.
Conventional weather forecasting starts with observational data about the actual weather and atmospheric conditions. These are fed into mathematical models of the physical processes involved, to predict how the weather might develop in the hours and days to come. When new observational data is available, the system is updated and a new projection made.
For cloud formation and development, these data typically come from conventional radar antennae, which might take five minutes to produce 15 vertical scans through a cloud formation. That is not much spatial detail to work with, and the five-minute delay is too slow to catch significant developments. “The evolution of the cloud just seems chaotic,” says Miyoshi.
So, the RIKEN researchers turned to another type of radar, called phased array. It can produce 100 vertical scans through a cloud in half a minute. “Thirty seconds is fast enough that we can really track the motion and evolution of rapidly evolving clouds,” says Miyoshi.
Wrestling the data
The next challenge was to synchronise this massive amount of data, updating very rapidly, with a physical weather model. This kind of data assimilation is Miyoshi’s speciality. “The whole workflow of the software needed to be designed very carefully, so that all the data coming in was moving around in a proper way,” he explains.
Then they held their breaths to see how the weather model would react. “We had no experience at all about how the model might behave if we inject this huge amount of data every 30 seconds.”
Initial work was carried out on RIKEN’s K supercomputer, but with a view to deploying it on its more powerful successor, then on the drawing board. A first trial covering Tokyo over the summer of 2020 ran on a research supercomputer operated by Tokyo and Tsukuba universities. This produced some successful predictions, but also some failures, and resulted in a number of adjustments to the system.
The Tokyo trial was repeated in the summer of 2021 – the Olympic summer in Tokyo, when more than a few people were watching the weather closely. It used RIKEN’s new, more powerful Fugaku supercomputer. According to Miyoshi, the greater computing power significantly improved the system’s performance. “This year we had a number of convective cloud events in Tokyo during that period. We are still investigating the results, but our preliminary analysis suggests that we did quite well predicting those heavy rains.”
While this project was successful as a proof of principle, it will not translate quickly to everyday use. Phased array weather radar is not widely available, and the supercomputers needed to handle the massive amounts of data are scarce and expensive.
One option for making the technique more accessible would be to replace the physical weather model with a machine learning system, which would require less computing power to run. “If the system can learn from the data and produce a real-time prediction mimicking the physical model, then that would be a very effective way to make this approach operational,” Miyoshi says.
In addition to rainstorms, the technique might also be applied to other rapidly developing local weather events, such as tornados. But the next challenge, and a common one in the drive to improve weather and climate forecasting, is to scale up.
“It’s important to predict each individual cloud for a last-minute response, such as evacuation, but at the same time we would like to know 12 hours in advance the chance of these extreme events,” Miyoshi said. “That requires a different scale, and combining the different scales is a grand challenge in meteorology.