Information scientist Kazuhiro Sakurada wants to use large numbers of medical records to predict who is high-risk for coronavirus
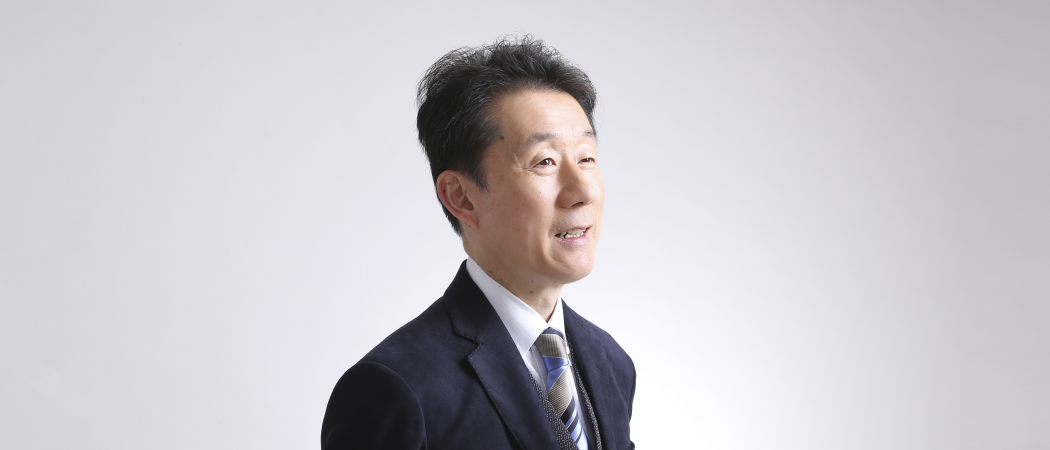
Kazuhiro Sakurada, Deputy Program Director at RIKEN. Photo: RIKEN.
Kazuhiro Sakurada, a senior researcher at RIKEN, Japan’s premiere research institute, speaks of illness philosophically. Symptoms develop over time, so “diseases are not the state of being ill, but the process of becoming ill,” he says. So how can he and other scientists forecast an individual’s state of health in the future, and address disease before it happens? By marrying biomedical knowledge with information science.
In an edited email exchange with Diane M. Fresquez of Science|Business, he describes some of his research at RIKEN’S Medical Sciences Innovation Hub Program, and how he feels a deep sense of responsibility to apply the programme’s know-how to overcome this pandemic.
Q. Tell us about your Covid-19 work.
Researcher: Kazuhiro Sakurada, Deputy Program Director Institution: RIKEN, Medical Sciences Innovation Hub Program, Japan Research area: Information science, AI, computer science, theoretical biology Key funder: Japanese Ministry of Education, Culture, Sports, Science and Technology |
Our project is to develop a procedure for using large numbers of medical records to predict who is at high-risk for coronavirus.
The SARS-CoV-2 infection ranges from asymptomatic to severe disease. A precise prediction model to identify high-risk people before infection would offer a potential way to exit efficiently from lockdown by isolating and vaccinating high-risk people.
Some background: Advanced age and comorbidities (multiple diseases/conditions) are major risk factors. But age and comorbidities are not enough to make precise predictions about who is high-risk for the coronavirus. Genetics is one powerful tool to find outliers such as elderly people resistant to illness and healthy young people with more-severe disease. But even identical twins can often vary in their susceptibility to disease and their physical aging. Therefore, susceptibility or resistance to COVID-19 has to be predicted by phenotyping: studying the physical characteristics of an organism as shaped by interaction between genes and environment.
Furthermore, the symptoms and outcomes of COVID-19 itself are complex. The neuro-endocrine system, probiotic bacterium and a person’s history of infection and vaccination influence the immune states of each individual.
Thus, we are developing “deep phenotyping” procedures for COVID-19, meaning that we study large data sets about individuals and key groups of individuals. Technically speaking, we have developed the concept of Phenotypic Information Geometry and the technique of Energy Landscape Analysis. The first aims to find clusters or similarities from multivariable datasets. The second is a technique to identify finite states of a person’s traits from big datasets.
A remaining challenge is to obtain high quality anonymised medical records before and after COVID-19 from a large number of people. For that, I am planning to collaborate with the Finnish Institute for Health and Welfare because, in Finland, a law has been laid down on the secondary use of health and social data. We need a data platform that can study data in high security and in accordance with the law. And for this purpose we are currently looking for funds.
Q. What were you working on pre-COVID-19, and how is it relevant to the pandemic now?
RIKEN Medical Sciences Innovation Hub Program (MIH) was established in April 2016 to develop cutting edge technologies and sciences for precise prediction and prevention of diseases using medical and health records. Now we have 42 members.
One of my colleagues, Eiryo Kawakami, has shown that Phenotypic Information Geometry is a powerful tool to predict clinical outcomes of epithelial ovarian cancer. There are only two states for the recurrence of that cancer: you either get it, or you don’t. But with COVID-19, we have to consider multiple states to predict the complex symptoms for the disease. This concept and procedure will be applicable to identify high-risk people before infection of SARS-CoV-2.
Another colleague, Tetsuo Ishikawa, has applied an energy landscape analysis to multifactorial time series data from a large number of people and obtained finite phenotypic states. This concept and technique could also be applicable to forecasting the risk of a person getting SARS-CoV-2 infection.
Q. What should be done to defeat the virus in your country?
More lockdown, plus find and protect high-risk individuals: At present only a few percent of the entire population of Japan has been infected by SARS-CoV-2. This indicates that a second and other waves will arrive in the near future. To ensure enough hospital and intensive care capacity, lockdown must take place again. In addition to the development of potential treatments, antiviral drugs and vaccines for COVID-19, a precise prediction procedure to find and protect the high-risk people is an important subject for study.
Precise prediction procedures for collective immunity: The development of collective immunity is estimated by the number of people who have antibodies against SRS-CoV-2. Part of the population would overcome infection by using both major types of immunity, innate and adaptive. But we have to consider the possibility that a lot of people prevent SARS-CoV-2 infection only by innate immunity, meaning the body never develops a “memory” of the disease. In this case, COVID-19 antibodies cannot be an indicator of resistance. I think that a procedure for predicting high-risk people from their medical records will contribute to understanding what kind of immunity we are dealing with.
Q. What is it like for you to work under lockdown?
I feel a deep sense of responsibility to apply our know-how to overcome this pandemic. I am now working in the field of AI, computer science and theoretical biology. Laboratories and offices are not necessary. I’m spending busy time working at home.