TalTech's Centre for Intelligent Systems is using AI to push forecasting forward
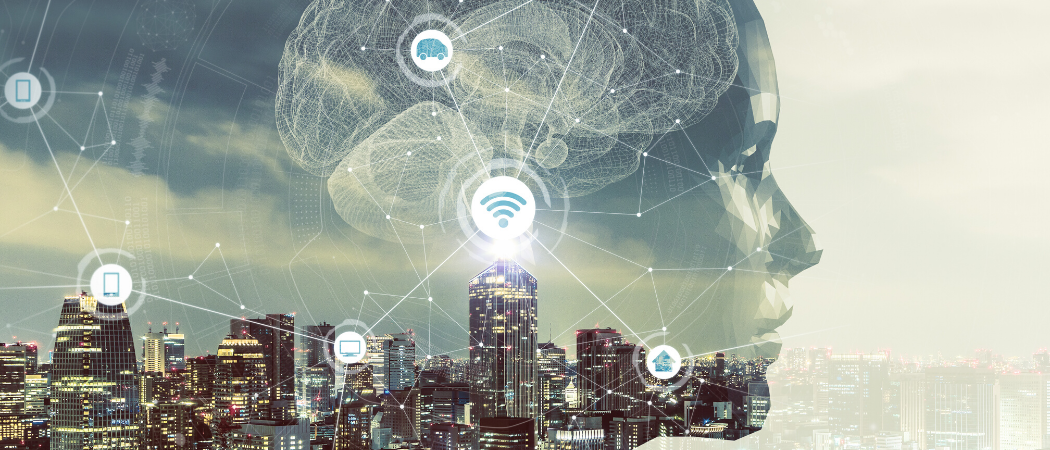
As more and more products consume electricity (think of all those gadgets you have plugged in at the moment), it is becoming harder and harder to accurately forecast power consumption. Power producers need accurate forecasts to fine-tune their plants to increase efficiency, reduce energy consumption and limit greenhouse gas emissions and pollution.
Arriving at such forecasts is made all the more difficult by the upheaval taking place in electricity markets with the expansion of the digital economy and the Internet of Things, and the growing reliance on electric motors in vehicles, bikes and scooters. “Electricity is increasingly the ‘fuel’ of choice in economies that are relying more on lighter industrial sectors, services and digital technologies,’” says the International Energy Agency (IEA).
Ironically, the digital economy could also be part of the solution: artificial intelligence (AI) and machine learning could help the power sector get a grip on fluctuations in demand. Employing sophisticated algorithms and control systems, the Centre for Intelligent Systems (CIS), based at Tallinn University of Technology, along with the university's Department of Software Sciences, has developed an electricity-consumption prediction model for the Estonian energy producer Alexela Energy SA. By analysing a vast amount of usage data, sometimes down to hourly consumption figures, the model accounts for possible changes in the number of Alexela’s clients, the fact that customers are distributed around Estonia and how temperatures vary in diverse climate conditions.
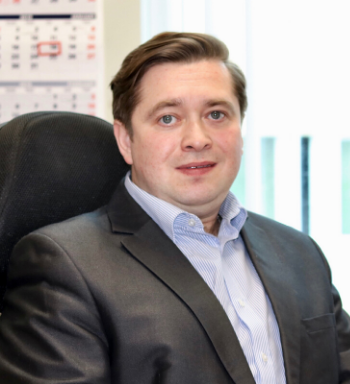
“It’s a dynamic process; it’s constantly changing,” says Eduard Petlenkov, professor of Intelligent Control Systems in TalTech’s Department of Computer Systems. “The conditions are always changing. Consumption is always changing; environmental conditions, weather conditions, everything is dynamically changing.” Dealing effectively with such dynamic conditions is one of the key goals of the systems the CIS is developing. It is trying to create control systems that can learn from data, adapt to rapidly changing environments and handle high levels of uncertainty.
Algorithms that can learn how to adapt
Uncertainty is nothing new in the energy industry, but advances in artificial intelligence and machine learning are paving the way for greater use of self-teaching applications and adaptive-control methods in the industrial world. Alexela and other power producers already use adaptive algorithms (think of sophisticated feedback loops) in their control systems, but in some cases the formulas are decades old, Petlenkov says. “The main problem is that these algorithms are hard to fine-tune. We want to replace these algorithms with ones that are more advanced and are able to learn themselves. And we have to convince industry to take this new technology.’’
The older generation of formulas “were complex mathematical algorithms and very specific for each process,’” explains Petlenkov. “We are trying to make systems to learn – to learn themselves and adapt themselves. This is much easier than designing a specific adaptation algorithm; this is the possibility to learn from data.’’
Indeed, advances in computer science and automation have given rise to the idea that the world is on a cusp of another industrial revolution. Enthusiasts have coined the term Industry 4.0 as shorthand for the advent of self-optimising production plants and supply chains.
Underpinning this concept is much greater use of connectivity. As more and more machines come online, reams of data are becoming available, just needing to be collected and used efficiently in order to improve the way control systems operate. “We have a lot of sensors, we get a lot of data and we have to make automatic decisions in industry,’’ Petlenkov says, describing the emerging data-mining approach for predictive modelling. ‘‘We get data more often’’ than ever before, he adds. “We get information maybe every second.’’ That flood of real-world, real-time data is used by the control system to make quick adjustments in a rapidly changing industrial landscape.
Tapping new forms of computational intelligence
The energy forecasting work with Alexela is part of a broader push by CIS to develop a new generation of modelling and control techniques by combining advanced algorithms with computational intelligence methods. It involves concepts, such as artificial neural networks, genetic algorithms, fuzzy logic and data-drive control design. As it designs control systems, the CIS uses virtual reality to get a better understanding of the complex processes involved, creating “digital twins” that can test out processes in life-like environments.
The Centre has also used its research to develop an AI-based system for the classification and estimation of insurance risks for Ergo Insurance SE. Moreover, the Centre’s algorithms have helped Skoda Auto to evaluate driver distraction caused by in-vehicle information systems. Some of the tools the researchers have developed can be downloaded from the MathWorks File Exchange, which sees about 2,500 downloads per year.
Set an objective and step back
The ultimate idea is to have the control systems learn from the data inputs and changing conditions so that human input is minimal. “Our task is to get rid of the influence of humans. A human should be involved as little as possible,’’ Petlenkov says.
But “the goal should be defined by a human; the goal cannot be defined by just any intelligence,’’ he stresses. “We define a criteria – what we want to optimize.” That objective could be, for example, to make the system faster, or more precise, or to reduce the consumption of resources; or to cut pollution and emissions. “But after the goal is defined by a human, other steps have to be automated,’’ Petlenkov says. “This is where we are going. This is what we are aiming for.”
Perhaps inevitably, the next-generation of tools for adaptive control systems will face some resistance in real world. “In fact, industry at the moment is very afraid of applying advanced algorithms’’ because of their complexity, Petlenkos says. “This is a kind of problem,’’ he says - one CIS is working hard to tackle.